Why Tech Roles Adopt AI Faster Than Others
“It’s not OK to leave any part of your business behind.”
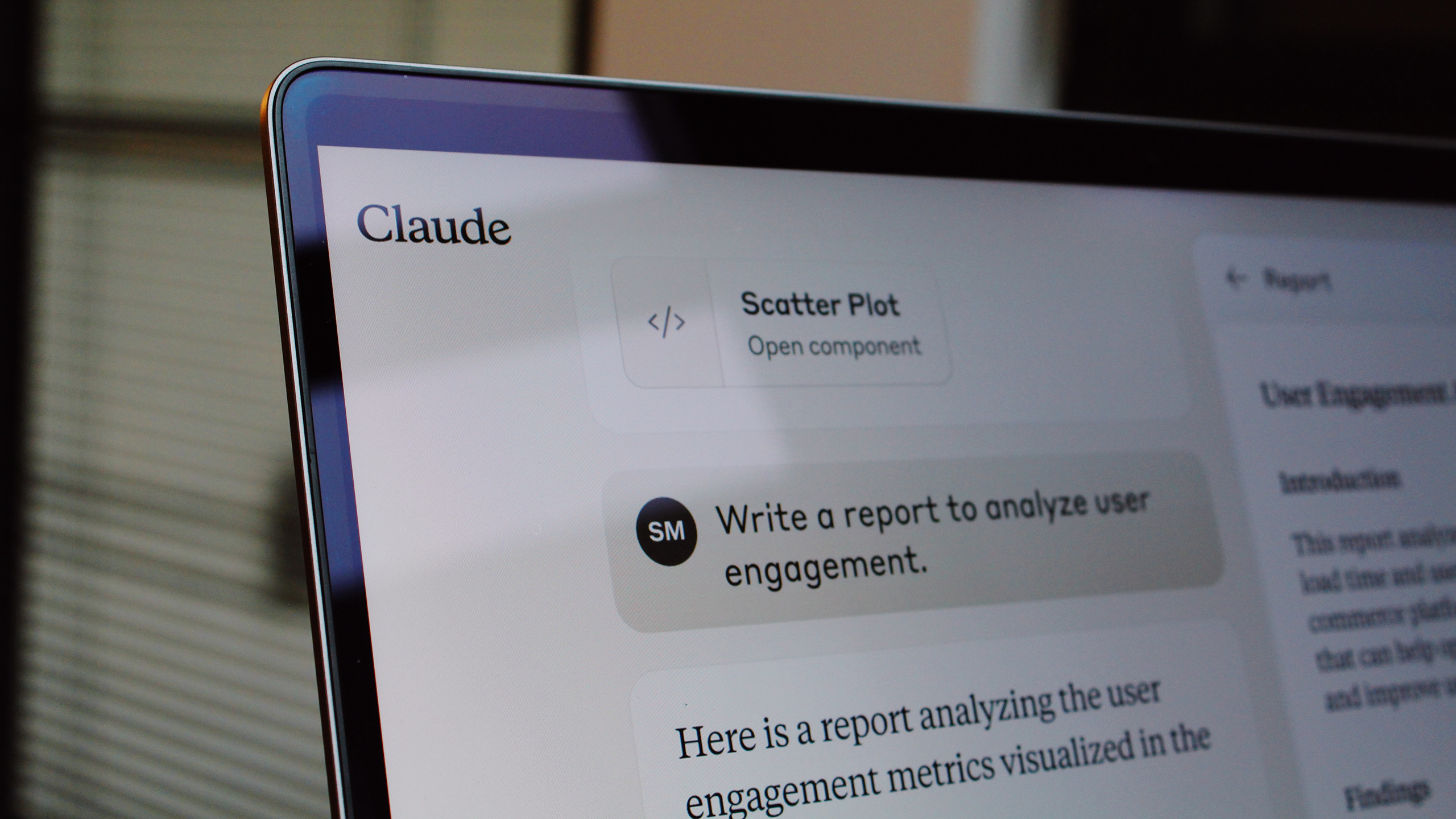
Sign up to get cutting-edge insights and deep dives into innovation and technology trends impacting CIOs and IT leaders.
AI is changing the way we work. But it may be changing some sectors more than others.
The Anthropic Economic Index, released by the model developer earlier this week, found that workers in the computation and mathematics field tend to use its AI models more than those in others. The report highlights that while some jobs more easily adapt to AI, other roles require a “nuanced” approach, said Julie Geller, principal research director at Info-Tech Research Group.
“It makes sense that it’s been adopted in this way … it’s making the biggest impact there,” said Geller. “But that doesn’t mean that it can’t make a big impact on a variety of roles.”
Anthropic’s index, which analyzed user conversations with Claude, its AI assistant, while preserving user privacy, found that more than 37% of users were using the chatbot for “computer and mathematical tasks.”
- Some of the top user groups include computer programmers and software developers of systems and apps, using the platform to develop, debug code or troubleshoot problems.
- AI models are a natural fit for collaboration with technical jobs, said Geller, assisting and speeding up things like code generation or user interface design. Developer copilots are an enterprise AI niche that a lot of tech giants have leaned into, including Google, Microsoft-owned Github, OpenAI and Amazon.
But adoption gets tricky once you look beyond the tech-heavy roles, said Geller. A lot of organizations are still navigating where the line should be drawn to implement AI into departments like marketing, sales and HR. “There’s so many different approaches that it becomes a bit more niggly on who drives what – how do we come together?” she asked. “How do we collaborate? How can we agree?”
And like any AI implementation, there are a few risks that come along with it. AI still faces data security issues, and using large, publicly available models with your workforce’s data could put certain information at risk. Additionally, there’s the risk of “overreliance,” said Geller.
“You can get into that mode of “set it and forget it,” or just copy and paste whatever output you’re getting from that agent,” said Geller. “And that can lead to some pretty bad decisions and sets the bar fairly low.”
However, as a lot of companies are struggling to see a return on their AI implementations, many are looking for broader, “end-to-end” applications rather than just leveraging it for technical roles, she said. A survey published last week by Informatica found that 97% of respondents are struggling to decipher the value of their organization’s generative AI initiatives.
Figuring that out takes more than just throwing AI at every department and seeing what sticks. Focusing on the ways individual departments can benefit and offering “equitable access” to the tech is vital “if you want to maximize impact across the organization,” Geller said.
The other important factor is education, said Geller. That means both creating a clear and consistent policy with employees on how they should (and shouldn’t) use AI, and teaching them how to leverage the models properly in the first place. Enterprises investing in upskilling programs to help employees “transition into AI-augmented roles and addressing the skills gap is going to be really important to integration.”
“It’s not OK to leave any part of your business behind, or any part of your workforce behind,” Geller added.