Happy Monday and welcome to CIO Upside.
Today: Though tariffs and export restrictions are pinching chipmakers and hyperscalers, a development slowdown may actually be helpful for enterprise adoption, experts said. Plus: Everything you need to know about neuromorphic computing; and why Intel might want to heat up data centers on purpose.
Let’s jump in.
Chipping Away at Big Tech’s Bottom Line
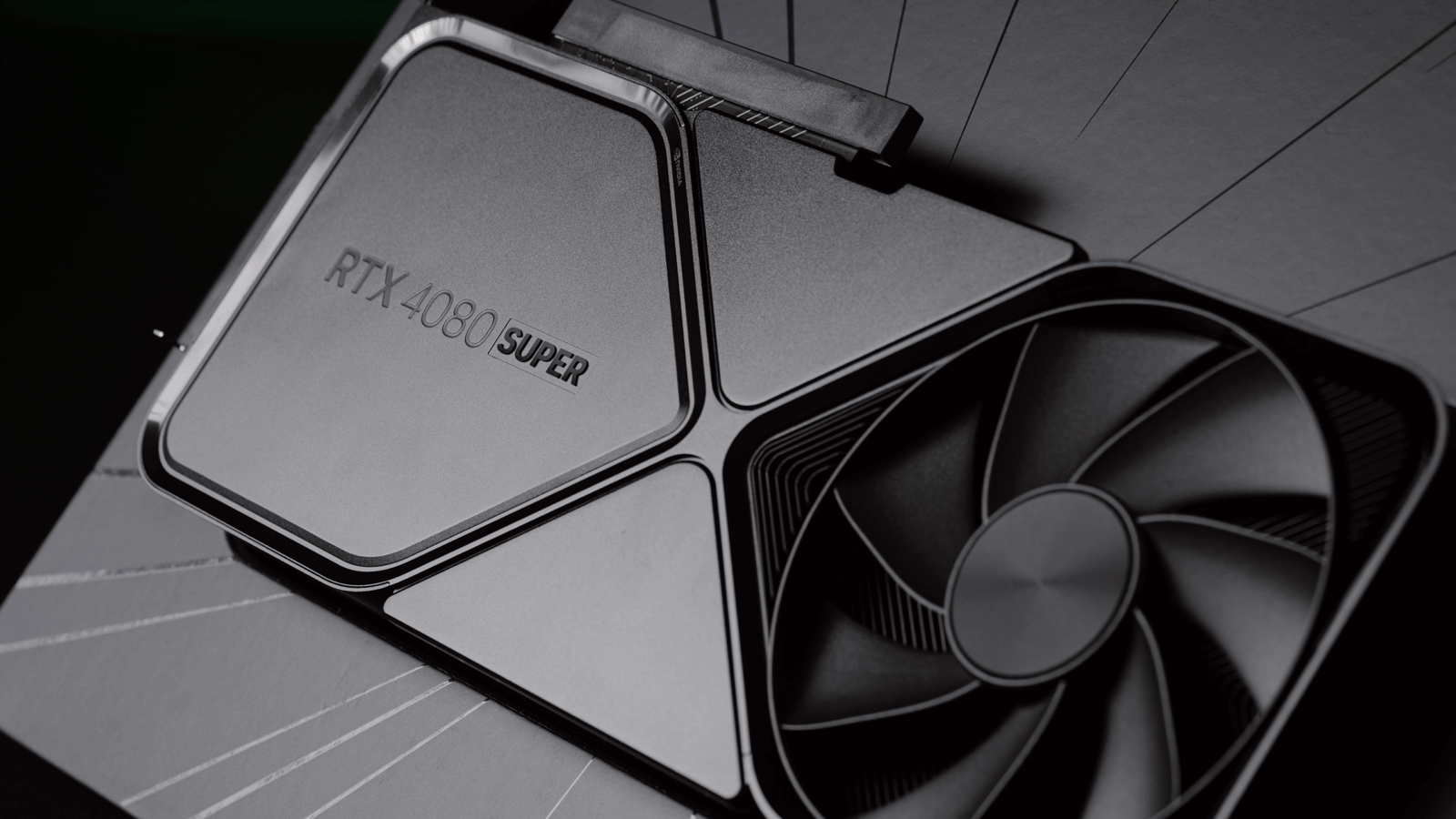
The Trump administration’s export restrictions and tariffs have put AI chipmakers – and the tech industry as a whole – in a precarious position.
Major U.S. chipmakers said last week that they would incur millions of dollars in charges because of a new export restriction — a requirement that they obtain government licenses to ship certain computer chips to China, permits that companies have no assurance will be granted.
With the new requirements, AMD claimed it will incur charges of up to $800 million related to its MI308 products, and Nvidia claimed it will record $5.5 billion of charges in the first quarter related to its H20 AI chips. Intel will also reportedly require a license to export some of its advanced AI chips.
Restricting the export of Nvidia’s H20 chips may end up “becoming a self-inflicted setback if it remains in place for too long,” said Tejas Dessai, director of research at Global X ETFs. “Cutting China off from state-of-the-art chips could ultimately accelerate domestic innovation and create more formidable competition for Nvidia.”
While AI chips have gotten a reprieve from widespread tariffs, it’s only temporary: Commerce Secretary Howard Lutnick told ABC News last week that tariffs on semiconductors are coming in “probably a month or two.” Nvidia may already be preparing for the impact, announcing this week that it aims to build $500 billion in AI gear in the U.S. by 2029 with a host of other partners.
- “I think there will be some development that occurs in the US, but I think it will be measured, and it will adjust based on how policies in the US are adapted and take hold,” said Scott Bickley, advisory fellow at Info-Tech Research Group.
- And chips aren’t the only things that go into building AI. Other components will also face tariff-related cost increases that hyperscalers will simply have to eat, said Bickley.
- “While the cost of building a data center may go up 5% to 15% … at this point, I’m not really seeing the investment materially change from the hyperscalers,” said Bickley.
The big question remains: How much of this chaos can enterprises weather? Businesses may be able to handle a good deal of pressure from the shifting economic currents, said Dessai. “For now, our view is that they can absorb much more than the market currently anticipates so that they don’t lose their lead,” he added.
And with the risk of a slowdown, some companies may pick up the pace of their AI investments, rather than stomping on the brakes, he said.
Given the sheer amount of AI infrastructure that has either already been built or is in the near-term pipeline, even if “everything came to a screeching halt” in the next two quarters, “there’s more than enough capacity, and what happens is companies will pivot into efficiency and optimization mode,” said Bickley.
Many enterprises may start attempting to get more out of what they already have, he added, which will allow them time to wade through the “avalanche of advancements” that have been thrown at them.
“Digesting all of that is, I think, already a challenge for enterprises,” Bickley said. “While they might not say it, I think (enterprises) probably welcome a little bit of a slowdown.”
Neuromorphic Computing May Make AI Data Centers Less Wasteful
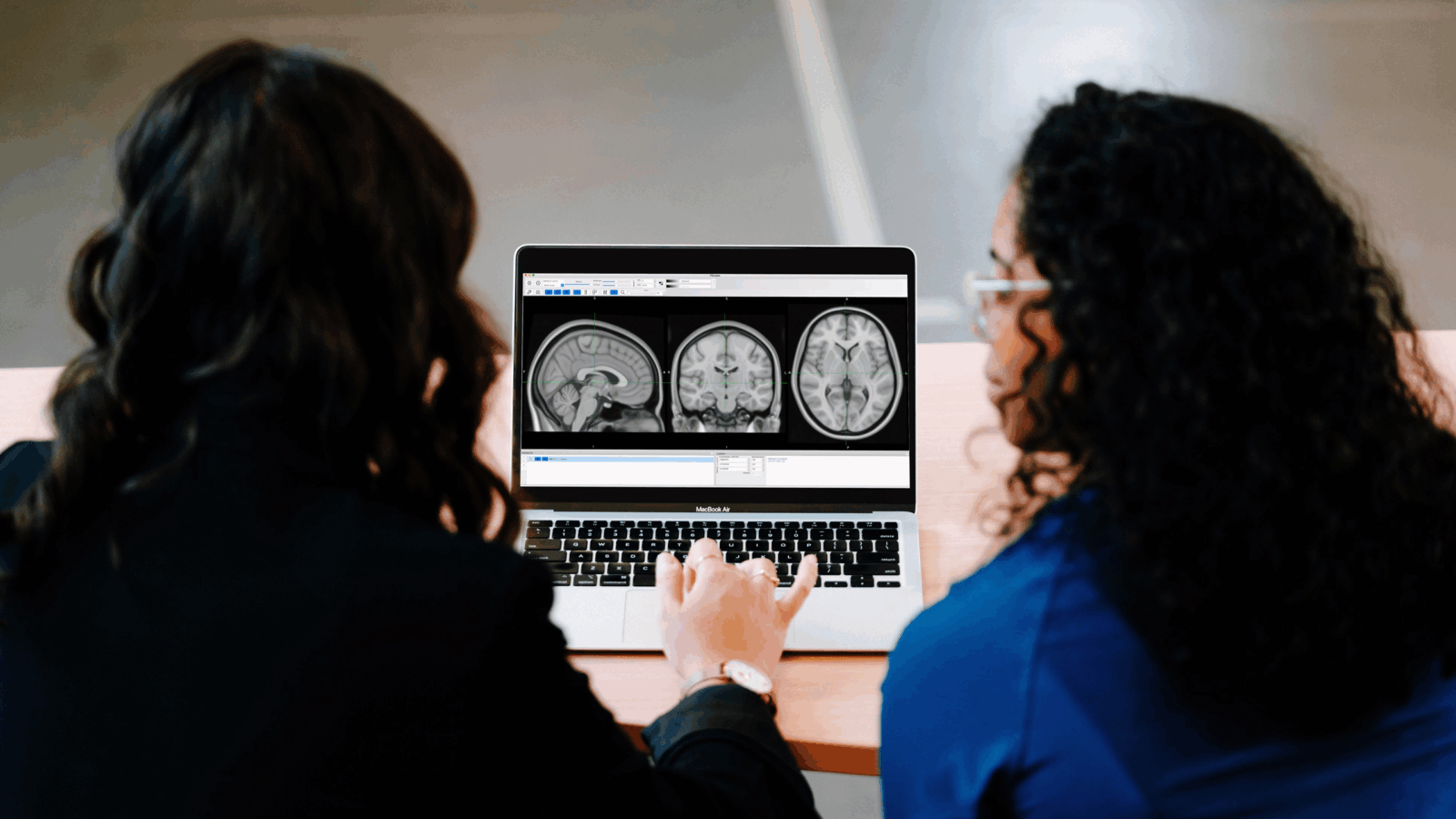
With AI driving up data center costs and energy usage, tech firms are increasingly interested in alternatives, including one that mimics the human brain: Neuromorphic computing.
Neuromorphic computing processes data in the same location in which it’s stored, allowing for real-time decision making and vastly reducing energy consumption in operations. The tech has the potential to help solve the power problem that continues to threaten the rapid growth of AI, said Sabarna Choudhury, staff engineer at Qualcomm.
Unlike conventional computing systems, neuromorphic computing leverages new hardware such as memristors – a combination of the terms memory and resistor – hardware components that handle processing and storing of data in the same location. That eliminates the energy-consuming processes of transferring data from one place to another.
“By offloading processing tasks to edge devices, neuromorphic tech can reduce data center workloads by up to 90%, enhancing energy efficiency and sustainability while aligning with carbon-neutral goals,” Choudhury said. This approach supports scalable AI without proportional energy increases.
It’s not just data centers that can reap the benefits. Any industry interested in AI stands to gain from the tech, Choudhury said, including aerospace, defense, manufacturing, healthcare, and automotive. Immediate use cases for neuromorphic computing are wide-ranging:
- In healthcare, neuromorphic computing is already being used to support real-time diagnosis and adaptive prosthetics. In the automotive field, neuromorphic vision sensors can provide improved safety.
- In industrial contexts, this tech can enable “real-time quality control and predictive maintenance in robotics, reducing downtime and energy costs,” Choudhury said.
- In military applications, such as DARPA’s SyNAPSE, these systems can be used in creating autonomous, low-power drones and surveillance tech in remote areas.
Neuromorphic computing is also catching the eye of major tech firms: The ecosystem of vendors includes the likes of IBM, NVIDIA, and Intel.
Intel’s Loihi chip, for example, can reduce energy usage by 1,000 times and enable real-time learning for AI deployment, Choudhury said. IBM’s TrueNorth chip also offers neuromorphic, energy-efficient architecture that processes information in real-time with minimal power consumption.
But challenges exist: New algorithms to integrate neuromorphic systems with AI or quantum systems need to be created. And beyond algorithmic integration, the success of neuromorphic computing depends on developing its full stack, from research and innovation in materials to new hardware architectures, circuit designs, and software and applications.
Existing gaps in the supply chain, however, open opportunities for new and established companies that want to innovate in a human brain-inspired tech that could pave the way to a more advanced, efficient, and sustainable AI future, said Choudhury.
“Neuromorphic computing is going to be a necessary step towards advancing AI,” Choudhury said.
Intel Patent Overheats Data Centers For ‘Energy Harvesting’
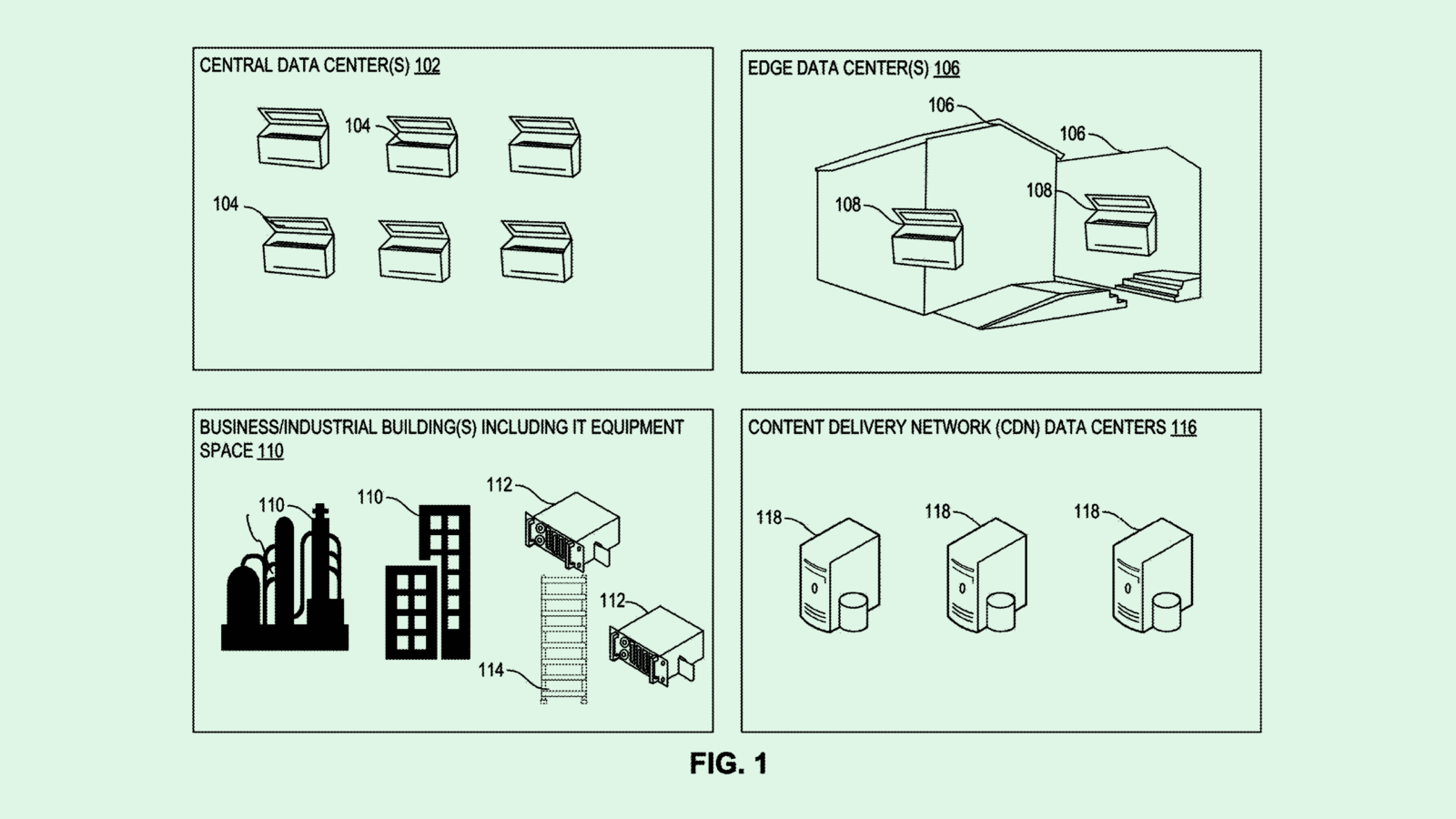
Data centers’ energy problem is only getting worse as AI and cloud capacity ramp up.
Among the tech firms in search of solutions is Intel, which is seeking to patent a system for “energy harvesting in data centers” that would generate power from center hardware such as server racks or individual CPUs and GPUs.
For each server rack, the system would monitor individual indicators, including power consumption, temperature, historical performance and cooling requirements. The system would then use those metrics to determine a “selection score” for the rack as a whole.
Instead of spreading out workloads to avoid overheating a certain rack, Intel’s tech would intentionally create hotspots based on selection scores, then harvest the waste heat for conversion into additional energy.
“As a result of inefficient and/or insufficient energy reclamation, opportunities to reuse the heat for purposes such as electrical generation remain untapped,” Intel said in the filing.
Intel is far from the first company to consider new methods to power up data centers: Microsoft has sought to patent ways to use cryogenic energy; Nvidia has researched ways to intelligently power down idle hardware; and Google filed a patent application for energy usage forecasting.
It makes sense that they’re looking to resolve this issue: The Department of Energy estimates that data centers will consume approximately 6.7% to 12% of total U.S. electricity by 2028. Worldwide, power demand from data centers is projected to more than double by 2030, hitting about 945 terawatt-hours – just above the energy consumed by all of Japan today, according to the International Energy Agency.
Though these inventions can help tamp down the data centers’ already outrageous energy use, they may be a bandage on a bullet hole given the sheer magnitude of current and projected AI and cloud computing demand.
Extra Upside
- Hallucination Happenings: OpenAI’s recently-launched o3 and o4-mini reasoning models hallucinate more than their predecessors.
- Disinformation Dump: The Trump Administration’s removal of cybersecurity guardrails has allowed for the spread of disinformation by a network of pro-Russian websites.
- Not a Sprint: More than 20 humanoid robots ran a half-marathon in China on Saturday, but couldn’t outpace their human competition.
CIO Upside is written by Nat Rubio-Licht. You can find them on X @natrubio__.
CIO Upside is a publication of The Daily Upside. For any questions or comments, feel free to contact us at team@cio.thedailyupside.com.