Happy Tuesday and welcome to CIO Upside.
Today we’re breaking down AI’s latest buzzword: Agentic. While this tech has caught the attention of the likes of Salesforce and Nvidia, one expert thinks this new wave of AI is “underhyped.” Plus: The pros and cons of synthetic data in model training; and Microsoft’s patents for cryogenic data centers.
Let’s take a look.
What Enterprises Need to Know About Agentic AI
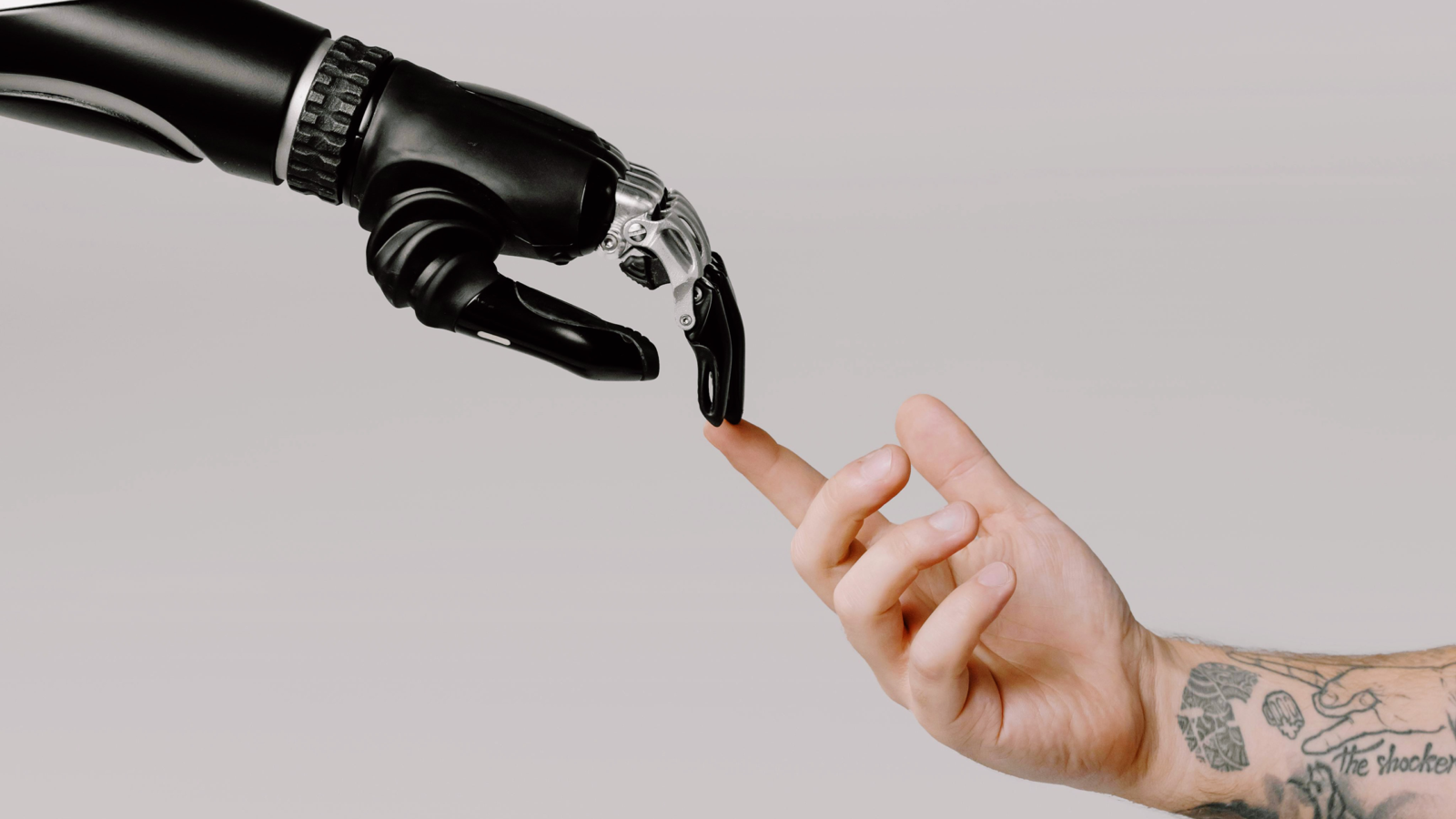
When a buzzword starts to fly around, its meaning sometimes gets lost in translation. With all the talk about AI agents, what does it really mean for AI to be agentic?
Agentic AI is the concept of giving an AI model the freedom to autonomously handle tasks, rather than prompting it over and over again until you get what you need from it. This new movement, which has caught the attention of tech giants and startups alike, may have the potential to put large language models to better use and upend business automation entirely.
The real value of agentic AI is present in the name itself, said Mike Finley, co-founder and CTO of enterprise AI firm AnswerRocket: giving the model agency. This works by giving models more control over the amount of time they’re allowed to spend on tasks, the tools and information they use to properly complete said tasks, and the ability to leave a task incomplete if they don’t have the capability to complete it, he said. “The reason that models hallucinate is because they’ve been forced to answer.”
Enterprises are quickly taking notice of this tech: In a November study, Deloitte predicted that 25% of companies that use generative AI will adopt agents in some form or fashion, with that number growing to 50% by 2027. And amid the buzz, a few big tech firms have made their commitments known.
- Nvidia’s Jensen Huang said in his CES keynote that “the age of AI agentics is here,” alongside announcing Nvidia’s own platform for building AI agents called Blueprints.
- Upon releasing Gemini 2.0 in December, Google called it the “AI model for the agentic era,” and Microsoft has expanded its agentic AI portfolio several times in recent months.
- And Salesforce made “Agentforce,” its platform for building and deploying AI agents, the highlight of its annual Dreamforce conference in September.
Tech giants’ interest here makes sense: Many are likely looking for a return on investment from the billion- and trillion-parameter generative models that they’ve spent the last several years honing.
While the original question-answer framework that those generative AI models have long followed led the tech to skyrocket with the dawn of ChatGPT, the capabilities of large language models – and people’s expectations of them – have grown rapidly in the last two years, said Brian Sathianathan, CTO of Iterate.AI. “Usefulness began to unravel itself … People are generally looking for the next level of problem solving.”
Agentic AI represents that next level, allowing users to spend less time being so-called “prompt engineers,” said Finley. And despite its quick rise to fame, agentic’s potential to shift enterprise automation may still be “underhyped,” he said. Rather than simply making jobs easier as many AI tools have promised, this tech has the potential to entirely automate job functions, he said.
“This thing can literally replace the way that you do business,” Finley said. “And the role that humans play is taking a step forward.”
Once agentic AI is further along, tech firms may even start offering “agentic employees for hire,” said Sathianathan. “Hypothetically, we can lease out an employee. It’s just like when a company’s outsourcing, there could be software, agentic leasing.”
But there are a few things enterprises need to remember before going all in on agentic AI. The first is knowing how to identify what is – and isn’t – agentic, said Finley. “If it’s not making decisions, if it’s not writing sequences of instructions, if it’s not using tools, if it’s not replacing workflows that people do, then it’s not agentic,” he said.
Next is to pick off the “low-hanging fruit,” said Sathianathan. If your enterprise is looking to adopt agentic AI, start by figuring out which use cases are the easiest to implement and start there, he said. After a few of those implementations, put together a comprehensive AI strategy to see where it may fit into the rest of your organization, he said.
Finally, remember that these models still may face the same problems as traditional generative AI ones – including data security, said Sathianathan. In fact, because these models are operating autonomously, there’s a risk that data security problems may be “amplified” with less oversight, he said. “There will be more standards, agent scanning and integrity capabilities that will come into the picture very soon.”
The Drawbacks of Synthetic Data in AI Development
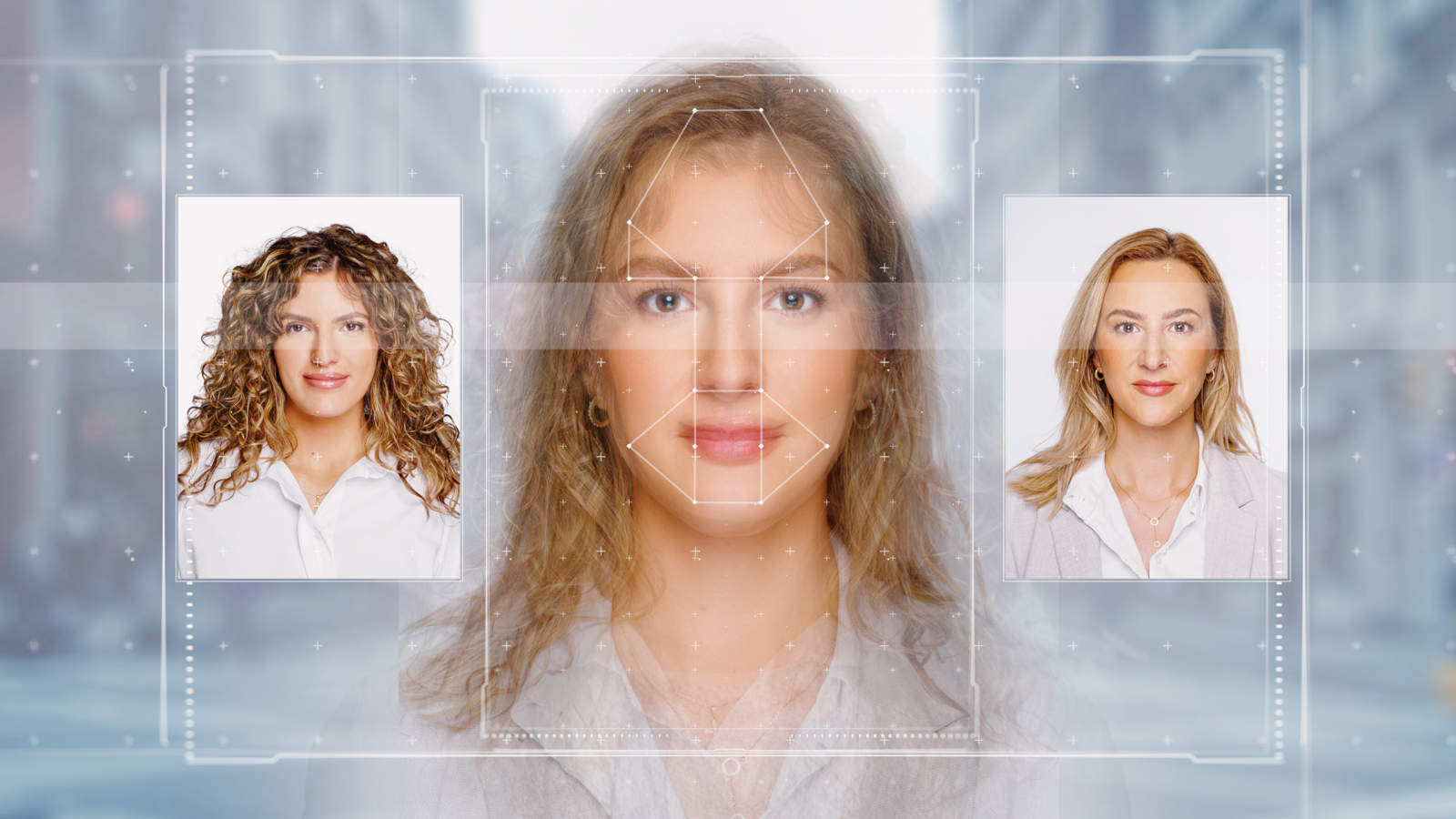
There’s no question that AI models are data hungry. But think twice before using synthetics to satiate them.
Synthetic data can be an incredibly helpful tool in the development of AI models – if used in the appropriate context. While the fact that this data isn’t based on real users or situations can help the data privacy issues that AI models often face, it has its drawbacks.
Let’s start with the good stuff. For one, it’s really easy to create good synthetic data that captures many aspects of the authentic data that it’s based on, said Bob Rogers, Ph.D., the co-founder of BeeKeeperAI and CEO of Oii.ai. That ease can be particularly helpful in contexts where regulatory hurdles using real data slow AI development, he said, such as finance.
“A synthetic set is a great way to make sure all the pieces connect,” said Rogers. “It’s not a panacea, but it certainly gets you past certain kinds of hurdles.”
However, while this data is “secure to a certain point,” it takes a “certain amount of sophistication to make a good synthetic set that captures the elements you need,” said Rogers. But the more sophisticated this data gets, the closer it mimics authentic data.
- Think of it like a sliding scale: On one end, you have synthetic data that’s not detailed and doesn’t closely display the features of the authentic data. While this synthetic data is safer to use, it’s usually not particularly useful, said Rogers.
- The other end of the scale, however, is synthetic data that so closely mimics its origins that it “basically exposes everything,” said Rogers. Though this is far more useful than less-detailed data, it raises security risks.
“It’s either not expressive enough to build good AI, or it’s so expressive that it could actually be risking security and it’s basically no better than anonymized data – which is also very easily re-identified,” said Rogers.
Even with these pain points, synthetic data has use cases. One big one is testing AI, said Rogers. For example, before training a model on real data, using synthetic data to “pressure test” how it may behave at scale could save a lot of time and effort if it ends up crashing, he said.
“Check the plumbing. Once the plumbing works, then you go into the real data and you build the models off that in a secure way,” he said.
But just because this data is fake doesn’t mean security can be any less rigorous, said Rogers. Whether it’s a chief data officer, a chief AI officer or just “someone who understands synthetic data,” the outputs of models that were built on this data need to be under surveillance for security slip-ups. Differential privacy, or a technique which ensures individual privacy within a data set, can provide an additional layer of security to synthetic data, he said.
“Train your people to at least know where the hot spots are,” said Rogers. “Not everybody has to be an expert in data security, but everyone should know when they’re treading on thin ice.”
Microsoft May See Cryogens as Key to Data Centers’ Energy Problem
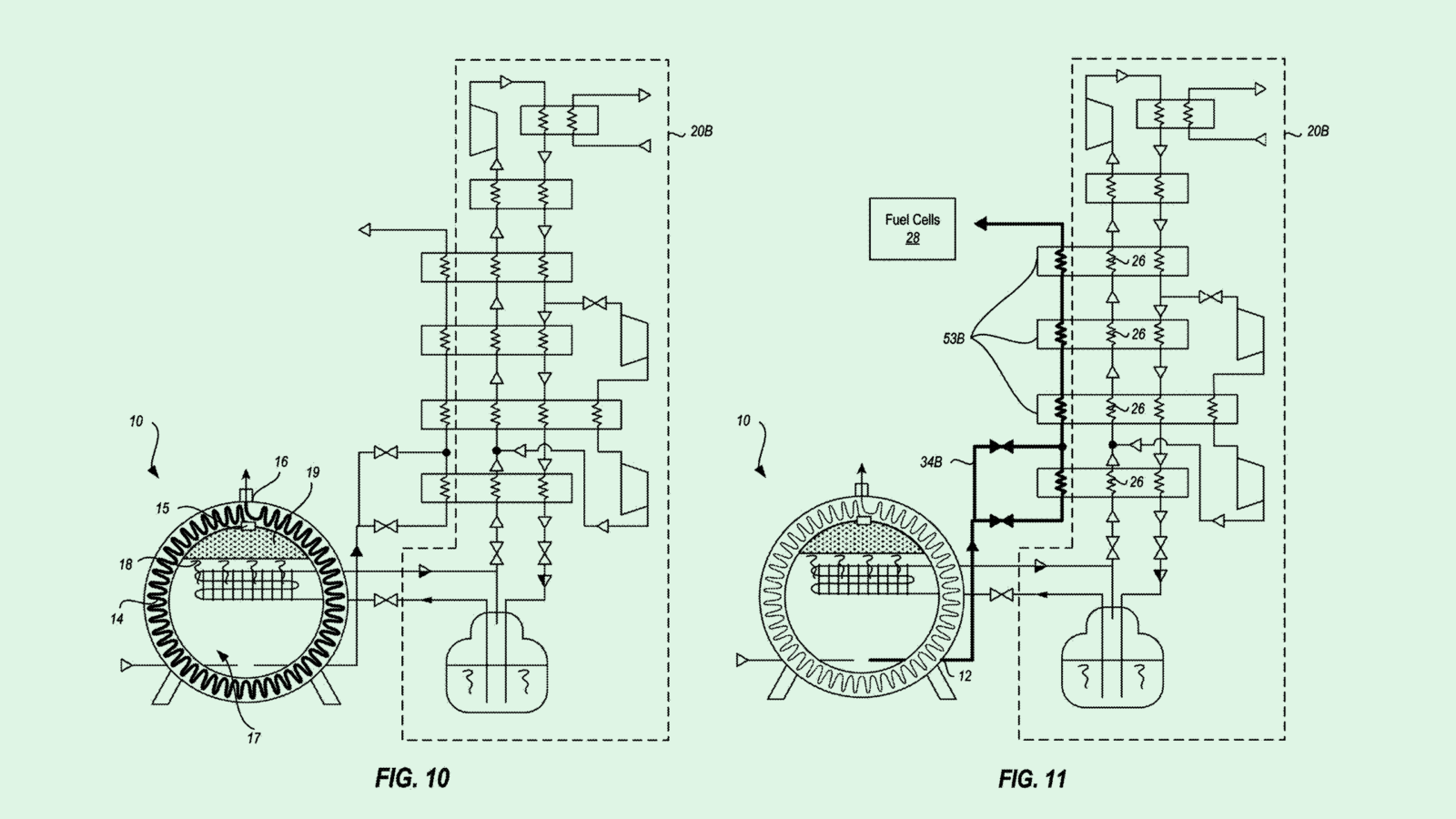
It’s no secret that data centers could cause major issues for the power grid. Microsoft’s patent history may reveal ways the company is looking to remedy that.
The tech giant is seeking to patent grid-interactive cryogenic energy storage systems with “waste cold recovery capabilities” that work in tandem with data centers. This tech aims to efficiently store and and manage cryogens, or substances that need to be stored at extremely cool temperatures, as a means of powering these server farms.
Microsoft’s patent details a system for cooling these cryogens down into a liquid form, passively storing them and using them as a backup power source. The system monitors the grid to decide when to use cryogenic energy, such as when grid energy costs are particularly high. The filing also describes a system for recovering waste cold energy, which essentially makes sure that excess cryogens aren’t lost in the process of converting them into a usable energy source.
“The use of fossil fuels in generating power is a known contributor to climate change,” Microsoft said in the filing. “Additionally, grid power is known to fail in extreme temperatures, such as extreme heat or extreme cold. Cryogenic power generation has been proposed as a potential power backup source.”
This patent builds on a previous Microsoft patent for grid-interactive cryogenic energy storage. These filings, plus another from recent years for cryogenic carbon removal, could signal that the company sees cryogens as a key to easing data centers’ energy problems – especially as AI threatens to worsen them.
A recent report from JLL found that the power demand on data centers is expected to double by 2029, largely fueled by the growth of AI and cloud services. While these companies likely aren’t keen to stop their AI ventures, many are looking for solutions.
Patents from other large tech firms target a similar goal, such as Nvidia’s patent to intelligently power down “idle cores” in data centers, Intel’s patent for clean energy budgeting, and Google’s patent to forecast carbon-intensive operations.
These firms are also seeking solutions beyond their patent portfolios. Google announced a deal worth $20 billion in December with renewable energy firms to generate carbon-free power for data centers. Amazon, Microsoft and Google also invested in nuclear power this year.
Whether or not cryogens are the solution to this problem, data center energy management appears to be top-of-mind for not only Microsoft, but every tech giant and data center operator that wants to keep making money from AI and cloud services.
Extra Upside
- DeepMind spinoff Isomorphic Labs expects research and trails for AI-designed drugs to begin this year, CEO Demis Hassabis said on a panel at Davos.
- Chinese AI firm DeepSeek claims that it’s recent reasoning model performs as well as OpenAI’s o1 model in some benchmarks.
- TSMC is confident that the company’s CHIPS Act funding will come in gradually under the Trump administration, CFO Wendell Huang told CNBC.
CIO Upside is written by Nat Rubio-Licht. You can find them on X @natrubio__.
CIO Upside is a publication of The Daily Upside. For any questions or comments, feel free to contact us at team@cio.thedailyupside.com.