DeepMind Patent Shrinks Model Size Without Compromise
Small and energy-efficient models have garnered growing attention in recent months.
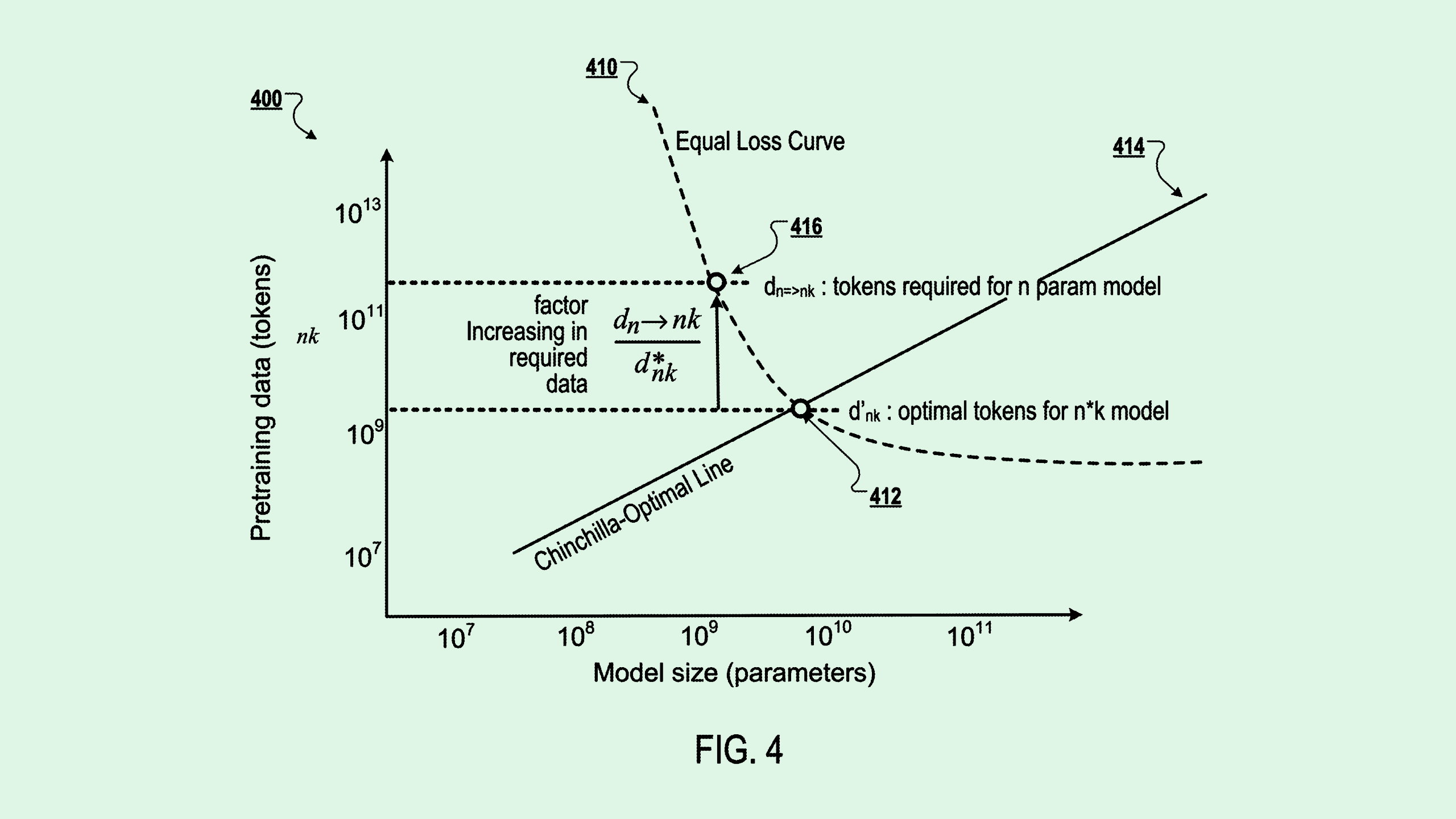
Sign up to get cutting-edge insights and deep dives into innovation and technology trends impacting CIOs and IT leaders.
AI training data isn’t “one size fits all.” DeepMind wants to figure out exactly what’s right for each model.
Google’s AI subsidiary filed a patent application for “determining training data sizes for training smaller neural networks using shrinking estimates.” To put it simply, the tech aims to figure out how much training data a smaller model needs in order to perform on par with a larger one.
DeepMind’s system does this with what the filing calls “model shrinking mapping,” which is basically a ratio equation to figure out how much data a small model would need by comparing it with a large model and its training data. That equation allows developers to figure out exactly how much data they need, avoiding overtraining and wasting resources.
“The second model will achieve substantially the same performance as the first machine learning model despite having lower computational cost, less latency, and a small environmental impact at inference time,” DeepMind said in the filing.
Though tech giants – Google included – have been fixated on making their large language models more powerful, small and energy-efficient models have captured increasing interest in recent months:
- Last week, nonprofit AI research institute Ai2 released a 1 billion-parameter model that it says can outperform similarly sized models from Google, Meta and Alibaba in certain areas.
- And Microsoft released Phi-4-Reasoning-Plus in early May, a 14 billion-parameter model built for logic-based tasks such as math and science. For reference, large language models tend to have parameter sizes in the trillions.
Small and large models each have their pros and cons. While small models are generally more energy-efficient as well as cheaper, they suffer in terms of capability and accuracy. Large models, meanwhile, are the opposite. DeepMind’s patent proposes a system for finding a middle ground, something of growing importance as enterprises seek efficient and cost-effective AI.