Microsoft Patents Signal Demand for Explainable AI
Because these models often operate in a so-called “black box,” many users have little to no understanding of them.
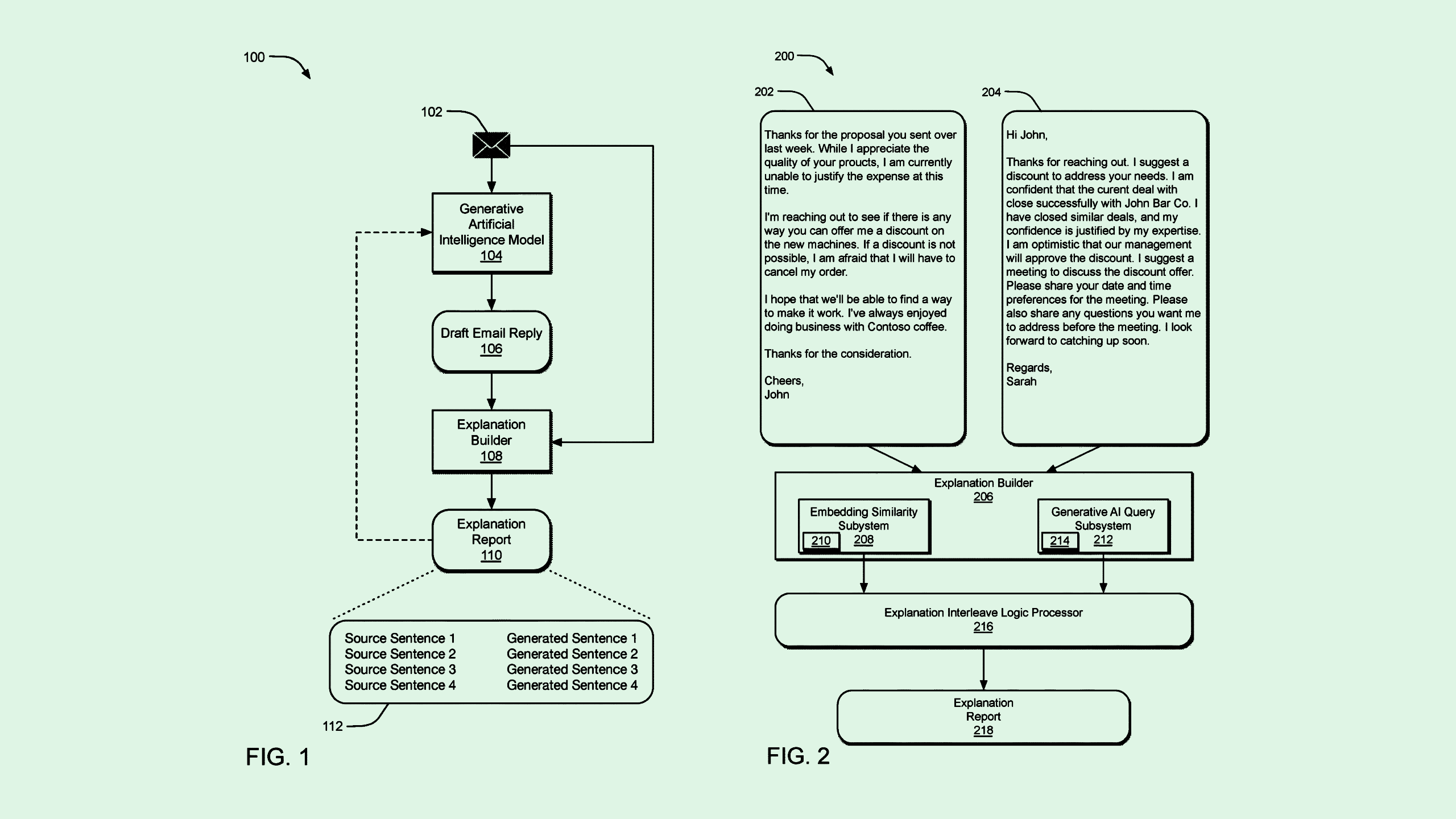
Sign up to get cutting-edge insights and deep dives into innovation and technology trends impacting CIOs and IT leaders.
AI can be complicated. But it doesn’t have to be.
Microsoft filed a patent application for what it calls a “machine learning model explanation builder,” that would help users understand where an AI model’s outputs are drawn from.
An explainable AI (XAI) system “is designed with the intent to help humans better understand how the AI system operates and arrives at its output,” Microsoft said in the filing.
Microsoft’s tech breaks down the input and output text into segments, or individual words and phrases, and compares the original query with the AI-generated response for similarity. That information is used to calculate similarity scores, which are then used to create a “map,” such as a side-by-side annotation, for users to understand where answers come from.
Additionally, Microsoft may be looking at ways to test the quality of its AI’s outputs: The company is seeking to patent a generative AI “output validation engine,” which basically ensures that an AI model’s output makes sense given the input.
Similar to the previous patent, the tech compares the raw data or query that was fed to the model with the output the model provided. This time, rather than being scored on similarity, the output is scored on overall quality, a rating that can be used in a feedback loop to improve the quality of future outputs.
Microsoft isn’t the only company to take on explainability: Oracle and Amazon have both sought to patent tools for explainable AI, and Boeing filed a patent for the concept’s applications in manufacturing. Together, they highlight a fundamental problem that AI adoption has faced: A lack of trust.
In a 2024 study from McKinsey, 40% of respondents reported that explainability was a key risk in adopting generative AI, and only 17% were working on methods to mitigate the risk.
Because these models often operate in a so-called “black box,” many users have little to no understanding of where their outputs are derived from. This can make it difficult for enterprises to rely on it and adopt it widely.
“We need to move AI from a black box to a glass box,” Kellie Romack, chief digital information officer of ServiceNow, told CIO Upside last week at the company’s Knowledge 2025 conference. “Help people understand it.”