Big Tech’s Fascination with Large Language Models May Be Overkill
A high-powered chatbot may not deliver the illusive dream of developing artificial intelligence that can teach itself.
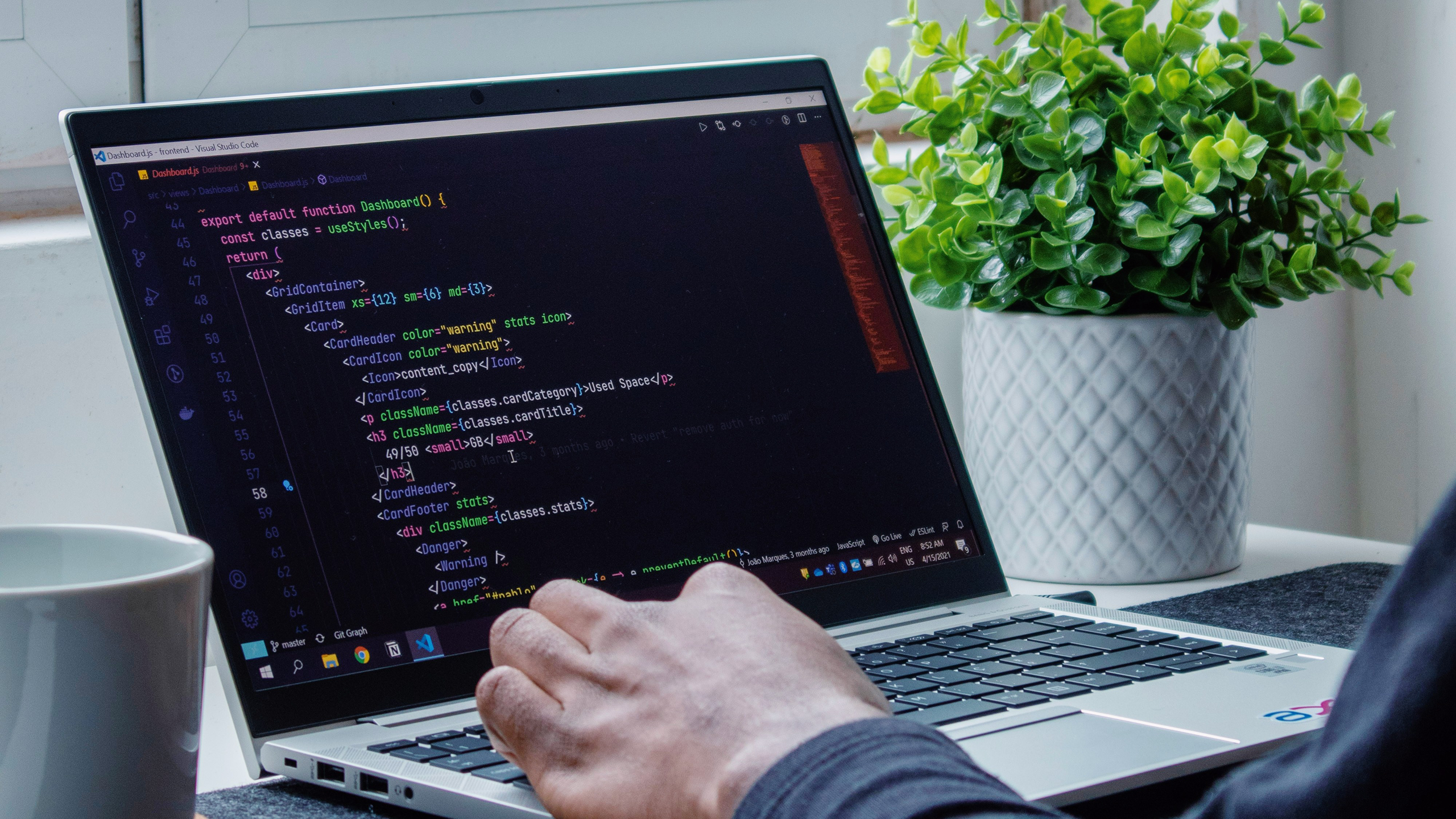
Sign up for smart news, insights, and analysis on the biggest financial stories of the day.
AI models are like babies: constant growth spurts make them fussier and needier.
As the AI race continues to heat up, frontrunners like OpenAI, Google, and Microsoft are throwing billions at massive foundational AI models comprising hundreds of billions of parameters. But they may be starting to lose the plot.
Size Matters
Big Tech firms are continuously trying to make AI models, well, bigger. OpenAI just announced GPT-4o, a massive multimodal model that “can reason across audio, vision, and text in real time.” Meanwhile, Meta and Google both introduced new and improved LLM while Microsoft readies its own, called MAI-1.
And these companies are sparing no expense. Microsoft’s capital spending jumped to $14 billion in its recent quarter – a number it expects only to increase. Meta warned that its expenses could reach $40 billion. And Google’s plans may be even more expensive: Google DeepMind CEO Demis Hassabis said it may spend more than $100 billion over time on developing AI. Many are chasing the elusive dream of artificial generative intelligence (AGI), in which an AI model can self-teach and perform tasks that it wasn’t trained for.
But such an achievement may not be possible with a mere high-powered chatbot, Nick Frosst, co-founder of AI startup Cohere, told The Daily Upside. “We don’t think AGI is achievable through (large language models) alone, and as importantly, we think it’s a distraction”:
- “The industry has lost sight of the end-user experience with the current trajectory of model development with some suggesting the next generation of models will cost billions to train,” Frosst added.
- Some AI experts agree. Yann LeCun, Meta’s AI chief and one of the godfathers of modern AI, told the Financial Times that large language models alone can’t achieve AGI, as they lack a “persistent memory,” have a “very limited understanding of logic” and cannot comprehend the physical world.
Bigger, Not Better: Aside from cost, massive AI models come with security risks and suck up tons of energy. Plus, after a certain amount of growth, research has shown that AI models can reach a point of diminishing returns. But making massive, do-it-all AI models is often easier than making smaller ones, Bob Rogers, PhD, co-founder of BeeKeeperAI and CEO of Oii.ai, told The Daily Upside. Focusing on capability rather than efficiency is “the path of least resistance,” he said. Some tech firms are already considering the benefit of going small: Google and Microsoft also launched their own small language models earlier this year – they just don’t seem to make the top of the earnings call transcripts.