Why Small AI Models Are Winning In Enterprise
The adage “go big or go home” may not always apply to AI.
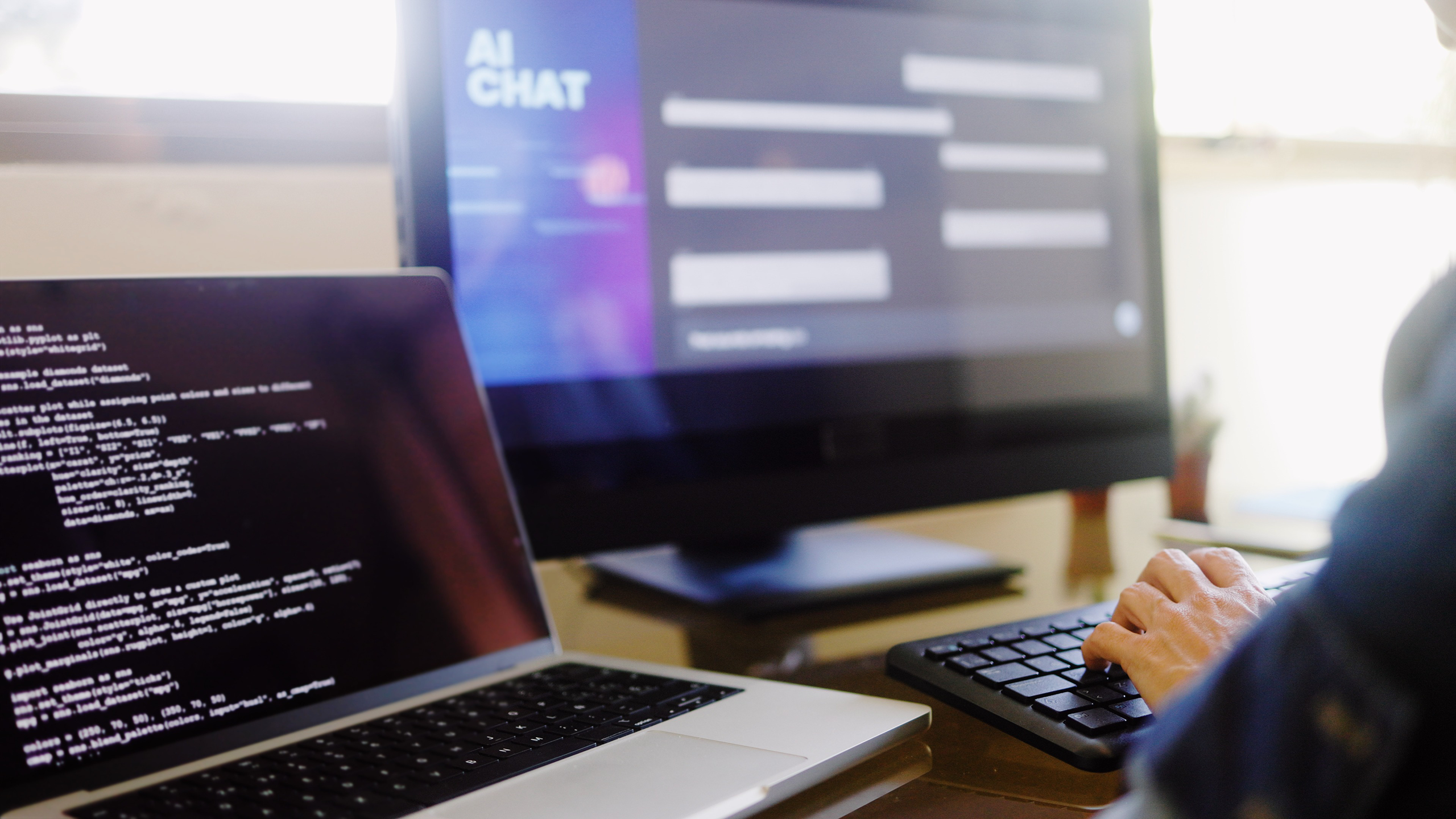
Sign up to get cutting-edge insights and deep dives into innovation and technology trends impacting CIOs and IT leaders.
Big Tech may be building even bigger AI, but it’s unclear how useful these massive language models are — or if, in fact, bigger is better at all.
More and more, enterprises are choosing small language models over their foundational, hundred billion-parameter counterparts. These models, which can be as small as a few million parameters, are often a faster, cheaper and more efficient alternative for enterprises that are still figuring out their AI strategies.
“Not every enterprise will have the infrastructure to operate [large language models] for every single need,” said Andrew Joiner, CEO of AI automation platform Hyperscience. “In a lot of ways, it’s like taking a helicopter to fly across the street.”
Most enterprises are using generative AI in some form or fashion. According to a survey of more than 500 IT leaders from Hyperscience and The Harris Poll, 79% of organizations reported using generative AI, and 97% said they plan to integrate it further in the next two to three years. But as it stands, these enterprises are still trying to grasp how generative AI fits into their operations – and many are still wary of the technology’s pitfalls.
- Data privacy remains a major obstacle to AI adoption: Around 47% of IT leaders reported privacy concerns as the primary barrier. Because of this, many are underutilizing the data they have available, with 3 in 5 organizations not leveraging their entire data estate.
- Integrating these models into core tasks in a meaningful way would mean giving them access to a company’s “most sensitive” data, such as legal contracts, purchase orders, user data and more, said Joiner. “They’re not appropriate to put into and use with a large language model.”
- Cost also remains an issue. Around 36% reported the high startup expenses made them wary of increasing AI integration, and 34% said high ongoing costs were a concern.
Thinking small may help enterprises solve these problems. With a small, locally run model, businesses have better traceability and data security control over what goes in and comes out of it, said Bob Rogers, Ph.D., the co-founder of BeeKeeperAI and CEO of Oii.ai. “You know exactly where your data is going, and that’s comforting,” he said. “For a lot of very sensitive data, local models are the right choice.”
Plus, with only tens of millions parameters to train as opposed to hundreds of billions, these models are far easier to put together quickly, are good for specific tasks, are more power efficient and can be more cost effective, said Joiner. That allows enterprises to “maximize their outcome” in building AI in the first place.
Enterprises seem to be aware of this benefit: Of the IT professionals who reported using generative AI in the survey from Hyperscience and The Harris Poll, three in four organizations said small language models outperformed large ones in areas such as speed, cost and return on investment. Tech giants, too, seem to see the benefits of going small, with Microsoft, Amazon, Meta, and Google all researching or releasing their own lightweight models in the past year.
But smaller models aren’t a panacea for IT’s AI woes, Rogers said. Simply put, these models are “not always as smart as the bigger models,” he added, and they tend to hallucinate more. They also tend to lack the people skills that large language models cultivate from billions upon billions of data points, Rogers noted, often making them less friendly to interact with. “They sometimes don’t have quite the level of nuance in their understanding of how ideas flow.”
That lack of nuance means that enterprise professionals have to pick and choose when small or large language models might be a better fit. While smaller models may not be the best choice for automating HR conversations or customer service interactions, they could be well suited for creating personalized AI assistants or helping with specialized research and development.
“You need to pick the right tool to get to the outcomes you need,” said Joiner.