IBM May Pay Closer Attention to AI Risk Amid Model Boom
The context in which an AI model is used can change how much risk it presents, and who it affects.
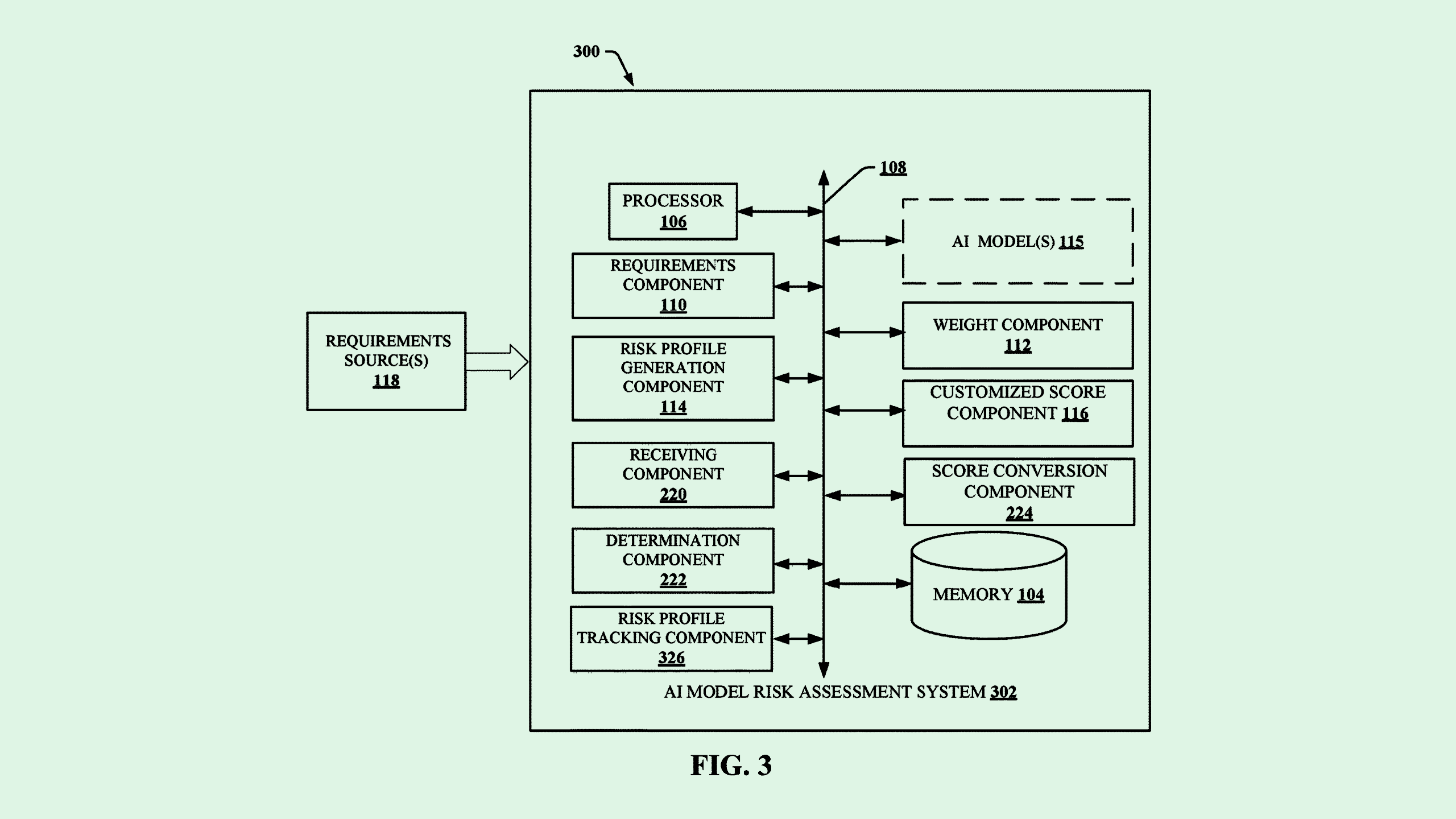
Sign up to uncover the latest in emerging technology.
AI comes with a lot of risks. IBM wants to track them down.
The tech firm filed a patent application for “providing and comparing customized risk scores” for AI models. IBM’s tech assesses AI models for several different types of risk depending on how they’re put to use.
“The consequences of a failure of an AI system can have substantial effects on individuals, organizations, or other entities that can range from inconvenient to extremely harmful,” IBM said in the filing.
IBM’s patent aims to provide “domain specific” risk assessments, adapting the scores to their specific intended use, governing standards, or other regulations. For example, IBM noted, a model used in facial recognition for policing may have stricter requirements and lower risk tolerance than one used in a general security system.
The context in which a model is used helps IBM’s tech determine which “dimensions” of risk it should focus on, such as fairness, explainability, or robustness, as well as the “metrics” by which to measure those dimensions, such as if a model’s risk factors impact different groups in different ways.
All of this information is used to calculate customized risk scores for each model, which are then converted to “comparable,” or standardized, scores. This enables stakeholders to compare the risk of their AI models across different applications, allowing for better transparency and accountability.
As AI development and deployment explode, so do the risks that these applications come with. The risks change depending on the context, and might entail data security, fairness and bias, or accuracy issues. And as IBM continues to focus on the enterprise AI market with data platforms and open source models built for business applications, paying attention to risk is crucial.
In enterprise contexts, models are often used for critical operations and applications in industries such as finance, healthcare, logistics, government, and beyond. Because of this, not understanding the risk that an AI model poses in its specific setting could present a huge liability.
“The difference between enterprise and consumer is the liability rests on the enterprise,” said Arti Raman, founder and CEO of AI data security company Portal26. “In a business-to-business setting, both the [organization] that is providing the model as well as the one that’s consuming the model care about risk and liability.”
And while the AI boom gave way to an initial flood of development, the return on investment for these products hasn’t quite hit the mark that the industry hoped for. That’s because “a large portion of experimentation is not going into production because of risk,” said Raman.
“Now that you’re seeing that this flow of investment isn’t quite getting you all the returns you wanted — not because the tech doesn’t work, but because the risk isn’t accounted for — you’re going to see a focus on risk,” she added.