IBM Diagnostic Patent Highlights AI-powered Healthcare’s Data Issue
AI has a lot of promise in the healthcare industry in areas such as documentation, imaging, and quicker diagnostics as staffing shortages loom.
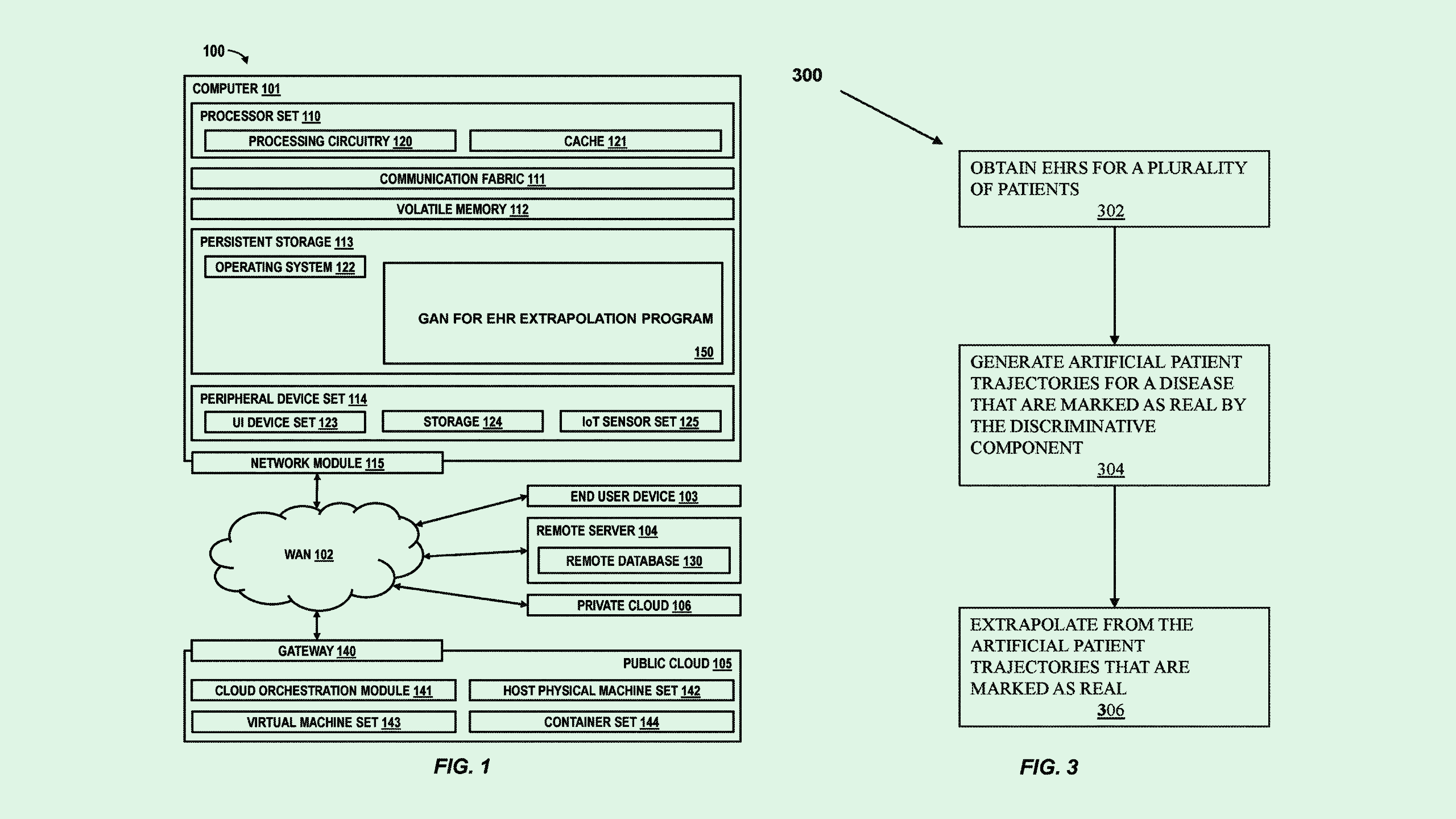
Sign up to uncover the latest in emerging technology.
IBM may want to help doctors make quicker diagnoses.
The tech firm filed a patent application for a way to use a generative adversarial network, or GAN, for “electronic health record extrapolation.” IBM’s filing outlines a way to train and utilize an AI-based patient diagnostic tool.
Current diagnostic models come with a number of problems, IBM said. Some are tedious and expensive to train, yet are “limited by disease rarity” due to limited training data, the filing notes. Others may have a wider net of training data, but yield results that are “often trivial and not-disease specific.”
To create a more robust diagnostic tool, IBM’s tech relies on a generative adversarial network, which uses two models — one to generate artificial data and another to discriminate between real and fake until the two are indistinguishable — to improve predictions. This network is trained on the authentic health records of real patients.
Once the network is trained, it can be applied to new patient health records, and predict both possible diagnoses of current conditions as well as “latent diagnoses,” which may appear later, based on predicted disease trajectory.
IBM isn’t the first to explore machine learning’s place in healthcare. Medical device firm Philips has sought to patent AI-based systems that predict emergency room delays and reconstruct medical imagery. Google filed a patent application for a way to break down and analyze doctors’ notes using machine learning. On the consumer end, Apple has researched ways to put the Vision Pro to use in clinical settings using a “health sensing” band.
AI has a lot of promise in the healthcare industry in areas such as documentation, imaging, and quicker diagnostics as staffing shortages loom. The problem, however, is the data. For one, in the US, healthcare data is strictly protected under the Health Insurance Portability and Accountability Act (HIPAA).
And given that AI needs a lot of high-quality data to operate properly, it’s particularly difficult to build models for healthcare settings. Though synthetic data may present a solution, because these models are being used in an area where accuracy is important, authentic data would likely allow for a more robust model — especially since lacking data leads to the dreaded issue of hallucination.
The other problem lies in how data is treated beyond training. Because AI has known data security problems, it may be difficult to get patients to trust models with their most sensitive information.