IBM Protects AI From Being Swayed by Bad Data
Its latest patent puts a magnifying glass to large wells of public data, aiming to make foundational models more robust against attacks.
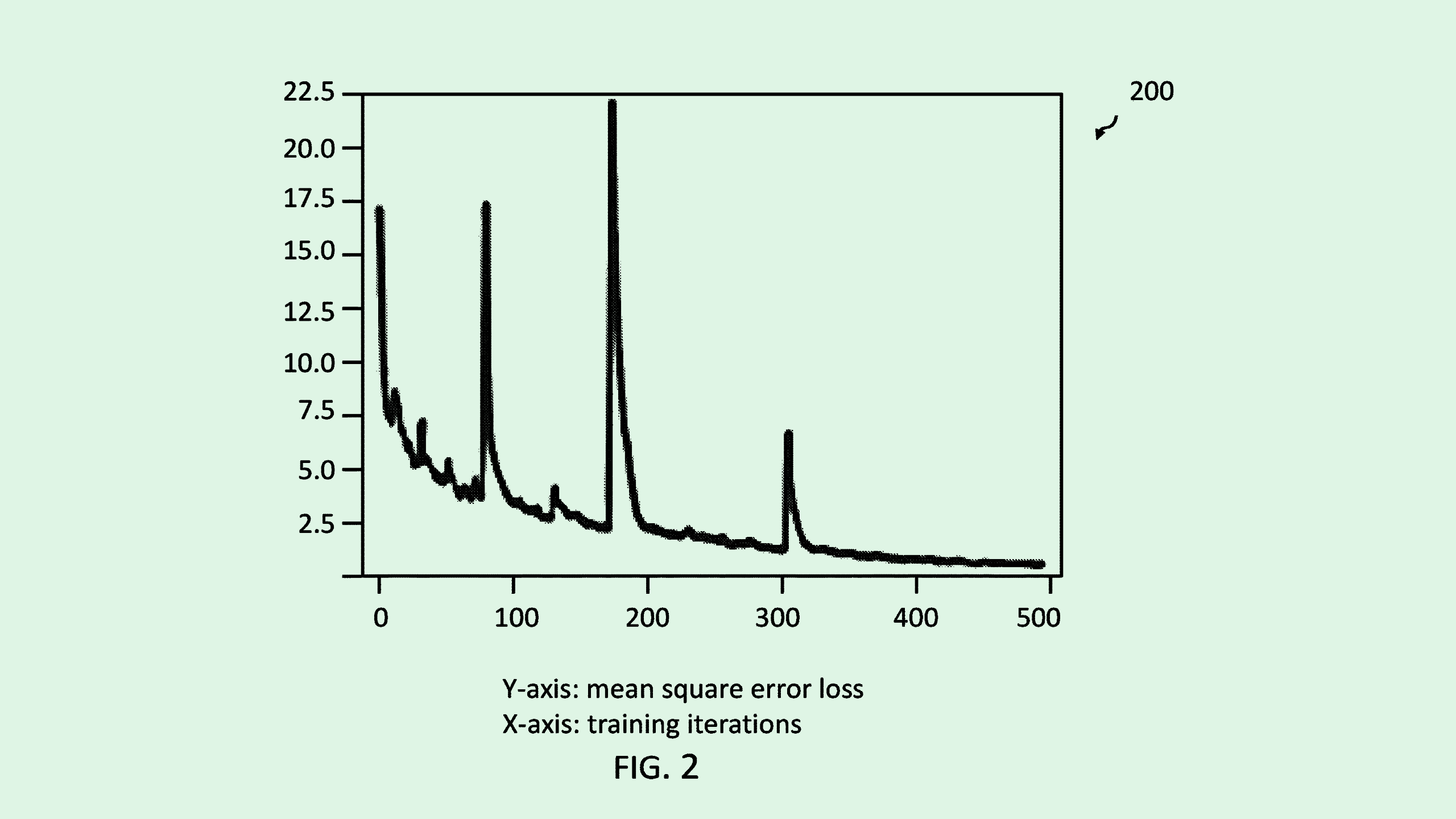
Sign up to uncover the latest in emerging technology.
IBM wants to keep its machine learning models from taking in junk data.
The tech firm is seeking to patent a system for protecting a machine learning model from “training data attacks,” IBM’s tech aims to keep AI models from sucking in public data that have potentially been compromised by bad actors.
“Attackers may manipulate the training data, e.g., by introducing additional samples, in such a fashion that humans or analytic systems cannot detect the change in the training data,” IBM noted. “This represents a serious threat to the behavior and predictions of machine-learning systems because unexpected and dangerous results may be generated.”
IBM’s tech aims to thwart this kind of threat by essentially applying a magnifying glass to the kind of data these models consume. To break it down: This system starts by initially training a machine learning model on a set of “controlled training data,” in which high-quality and reliable data is carefully selected for the task at hand.
After the first round of training, the system turns to the larger pool of public data to identify which of it may be “high impact,” or which data points may have a larger sway on the model during training.
Once the system identifies those data points, it creates an artificial “pseudo-malicious training data set” using a generative model. This set of data will essentially train the model on what attacks it may expect, so that it “may be hardened against malicious training data introduced by attackers.”
Data is basically the DNA of an AI model: Whatever it takes in is fundamental to what it puts out. So if a model is taking in tons of bad data – particularly data that may have a high impact on the model’s performance – then the outcome may be an AI system that spits out inaccurate and biased information, said Arti Raman, founder and CEO of AI data security company Portal26.
While smaller, more constrained models may not grapple with this issue, large foundational models that suck up endless amounts of data from the internet may struggle with this. And since the average consumer uses free and publicly available models, like ChatGPT or Google Gemini, over small ones, “It’s almost the responsibility of the model provider to make sure that those models don’t go off the rails,” said Raman.
“It’s pretty important to make sure that we have stable technology when it’s available to large populations,” she noted.
IBM’s system provides a way to defend large models from being tainted by a well of bad data, essentially acting as a vaccine against training data attacks. This method essentially builds “guardrails” into the model itself, allowing it to detect and fight against bad data when it comes across it, Raman noted.
Whether using this tech on its own models, licensing it out to others, or both, gaining control over patents related to strengthening foundational models could help IBM solidify its place among AI giants. “Everybody wants to figure out how to make models not misbehave,” said Raman. “So I think filing a patent is a pretty smart thing.”