Meta May Turn to Synthetic Data to Feed its AI Growth
Meta might want to make synthetic data from your Facebook posts. While this helps solve the privacy issue, the issue of bias is still present.
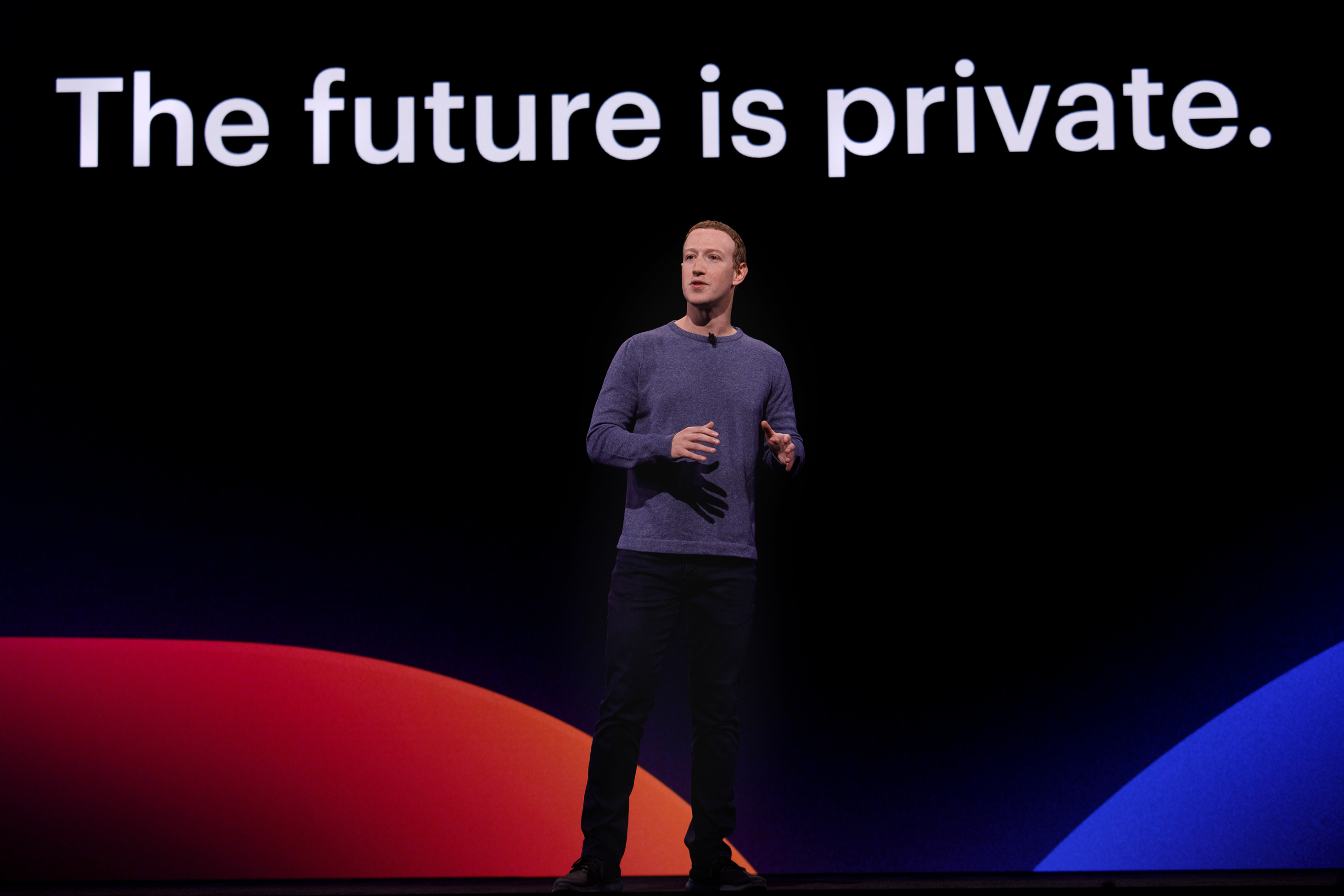
Sign up to uncover the latest in emerging technology.
Meta’s ambitious AI work likely eats up lots of data. The company may be looking to synthetics to fill that need.
The social media giant is seeking to patent a system for “generating synthetic data.” As the title of this filing implies, Meta’s system creates what it calls “simulated data” to help design custom-tailored applications without relying on actual system data, as to not sacrifice privacy for “high fidelity” design.
First, Meta’s system retrieves what it calls “data patterns” of content from a system or network (i.e., a social network). The system could glean these data patterns from user-generated content, including profile information and public posts such as “location information, photos, videos, audio, links, music” and more.
Data patterns essentially are the characteristics of actual data, but not the data itself, aiming to “preserve certain statistical characteristics associated with the actual content” that may be vital to application or hardware development. The system also determines “indices” associated with the data patterns, or essentially a way to quantify, describe and organize them.
Finally, the system uses a statistical model to generate “simulated data” associated with the content. Because the data mimics the characteristics and qualities of actual system content, this data can be used for optimization while “minimizing privacy risks.” And because the data is synthetic, it’s more easily shareable for development purposes outside of the system or network that it came from.

Meta has been quite intense in its endeavor to stake a claim in AI. The company is embedding chatbots into all of its platforms, using AI to build its metaverse and reportedly working on a large language model that’s several times more powerful than Llama 2, its commercial product. But training AI takes a lot of data — and Meta doesn’t have the best reputation for protecting user data.
While this patent is somewhat vague about how this simulated data could be used, synthetic data could solve the data privacy issue in AI training. But synthetic data needs to represent real-world data very well to actually be useful when training AI, said Molham Aref, CEO of AI coprocessing company RelationalAI. If your generator puts data “on a bell curve,” he said, you’re going to end up with a lot of average data that doesn’t represent “unusual circumstances that you can see in real-world data.”
“The trick is, if you’re not good at generating synthetic data that reflects the real world, then you’re going to have [AI] learn something that’s just fiction, because the synthetic data generator isn’t a reflection of the real world,” he said.
Plus, the bias problem with AI training data still exists when it’s synthetic, he said. If synthetic data is modeled on authentic data that contains biases, it’s going to reflect those same biases into the model. Aref gave Facebook as an example: While the synthetic data modeled from Facebook posts may be representative of older populations, it won’t reflect younger demographics as well.
But this isn’t a problem that only Meta is facing, said Aref. Most companies developing AI are seeking to do so in a way that doesn’t sacrifice privacy and doesn’t replicate biases, and many are turning to synthetic data as the solution. (That said, many companies are working on synthetic data solutions already, so whether or not Meta’s method is distinct enough that it can actually secure this patent is undetermined.)