Google Patent Trades AI Capability for Efficiency as Ambitions Mount
Google’s recent patent to boost AI efficiency signals that massive models may cost more resources than they’re worth.
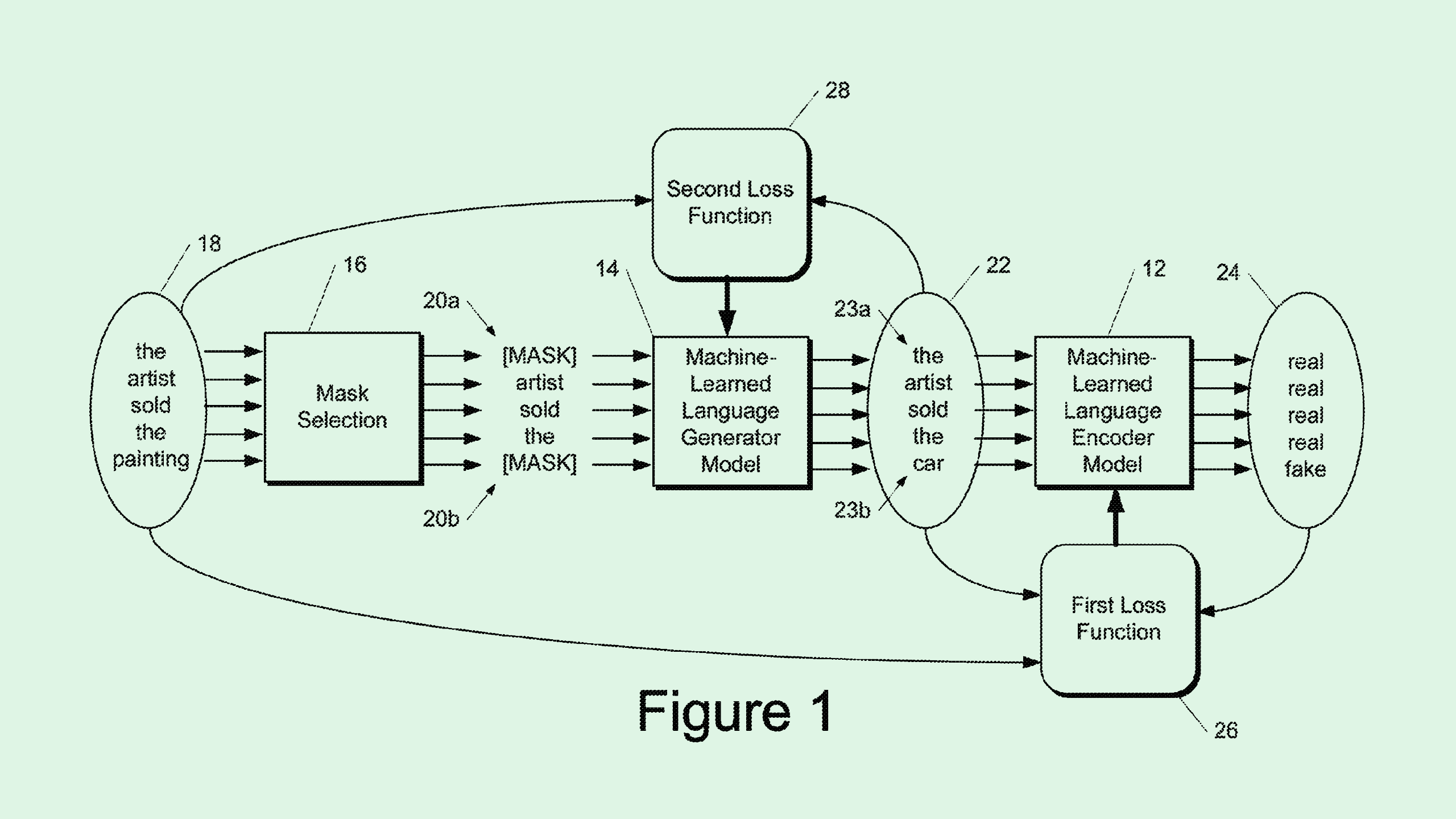
Sign up to uncover the latest in emerging technology.
Google may be looking for ways to cut down its AI power bill.
The company filed a patent application for “contrastive pre-training for language tasks.” The goal is to provide an alternative to “masked language modeling” approaches that typically suck up a lot of energy when training language models.
“An important consideration for pre-training methods should be compute efficiency rather than absolute downstream accuracy,” Google said in the patent.
When using masked language modeling for training, models guess a missing (or “masked”) word based on the context of the surrounding ones. These approaches typically mask a small subset of words — around 15% — in a set of data to teach the model to recover those missing words.
Conversely, Google’s method of contrastive training helps language models differentiate inputs from other plausible alternatives. Similar to masked language modeling, at the start of the process, a certain subset of words are masked prior to training. Then a “generator” model creates replacement words for the masked ones.
Finally, the main model is trained to predict whether the word in the sentence is real or AI-generated, helping it understand language better. This method is faster, more cost-effective, and more energy-efficient, as the objective is simpler than that of masked modeling, and the model only has a small subset of data to work on.
Google’s I/O event last week was packed with announcements, from AI search overviews to image analysis tools to AI personal assistants. Many of these tools were backed by Gemini, the company’s massive foundational AI model. But despite tech firms’ lofty promises about AI capabilities, models like Gemini are often simply too big for the average person or company to get consistent use out of them, said Vinod Iyengar, VP of product and go-to-market at ThirdAI.
Using a model like Gemini for every AI-related task is costly in both computing power and cash. Plus, many tasks don’t require the power that Gemini has, he noted. Infusing massive models into simple operations is like using an ax to cut a tomato.
So why would tech firms pour millions into these models in the first place? The simple answer: Because they can, Iyengar said. AI models with billions of parameters help companies like Google and OpenAI show that they have the “best in class” as a way to draw customers, he said.
However, those customers will likely end up using smaller, resource-efficient, and lower-latency models offered by these tech firms. “It’s a capability demonstrator,” said Iyengar. “But there is no real use case because people for the most part are going to use those smaller models.”
Patents like these show that the tide is shifting toward these models, said Iyengar. Despite the incredible capabilities of something like Gemini, the mounting cost of AI development will shift the paradigm from wanting the best to wanting “good enough” at the best price, he said.
“They’re kind of signaling to the market two things: We have the capability to build the most monstrous model that you can imagine, but we also have these great tier two models, which are very fast and very cheap,” said Iyengar.