Adobe’s Latest Patent Turns Language Models into Middlemen
Niche models could be more accurate, save energy and present less security risk than an all-knowing large language model.
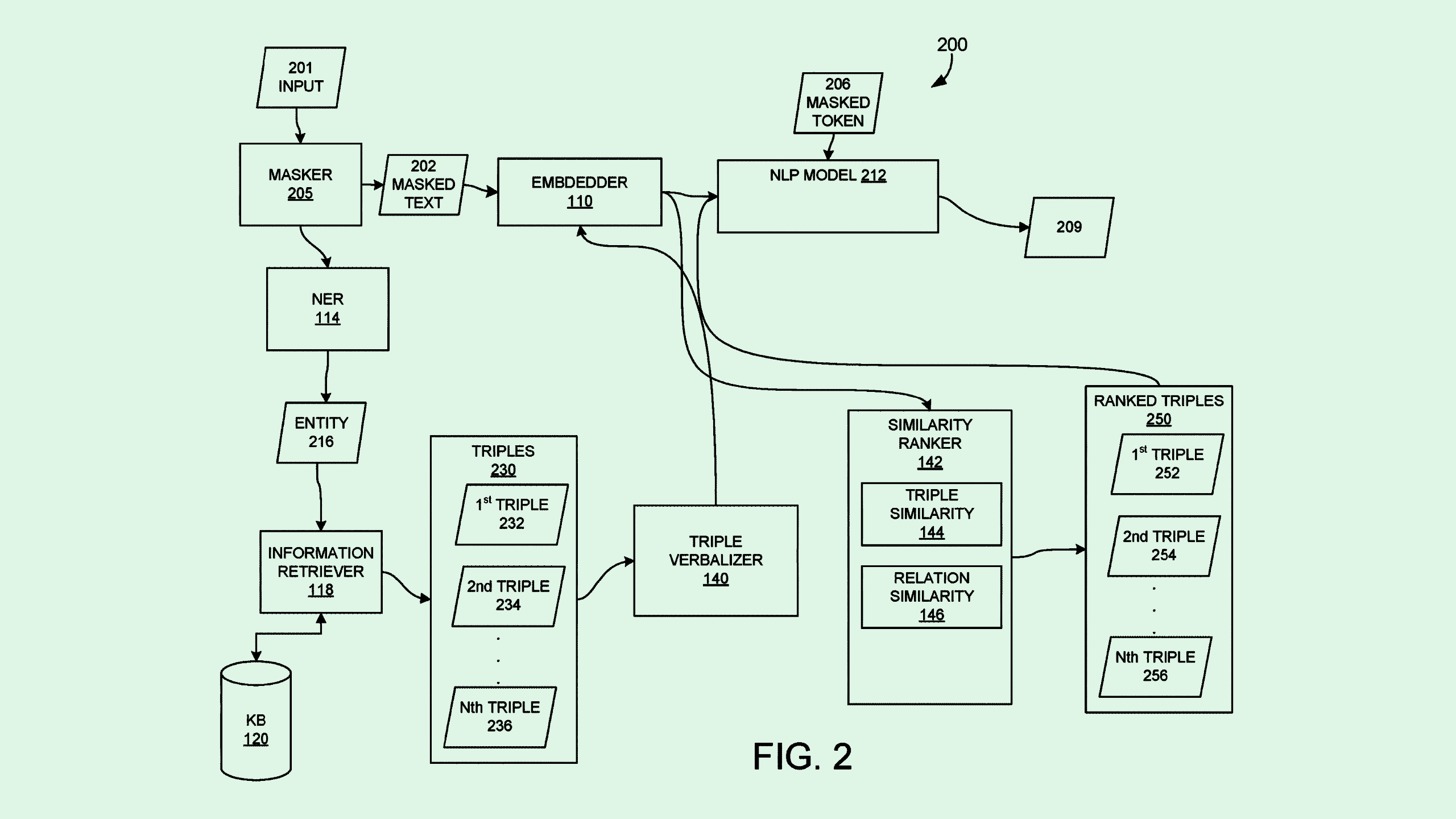
Sign up to uncover the latest in emerging technology.
Adobe doesn’t want its AI models to know the facts – just where to get them.
The company is seeking to patent a large language model that works with an “external knowledge base.” Adobe’s system uses an AI model as a middleman: Rather than storing facts themselves, it simply pulls them from a large database to make its predictions.
“Retrieving relevant information from the knowledge base and allowing the (natural language processing) model to use the information when making a prediction reduces the need for the NLP model to store facts,” Adobe said in the filing.
Adobe’s system first searches for and extracts the different “entities,” or subjects such as names, dates, or quantities, mentioned with a natural language sentence. The system then creates a modified version of the sentence through a process called “masking,” which essentially blurs out one of the entities named in the query to prevent the model from overfitting to the data.
The system then takes this information to scour a “knowledge base,” which can be any database that stores a larger corpus of information than an AI model would be able to (for example, Adobe noted, Wikipedia). The system then searches for relevant “triples,” which include three descriptors of the entity: subject, relationship, and object, to give the model more context. Adobe’s tech then ranks these triples based on similarity to the original sentence, selecting the most relevant ones from the bunch.
All of that information is taken back to the model, which is trained to make a prediction or response based on a user’s specific query.
Adobe’s patent highlights that the future of AI models may be smaller than we think. While Big Tech continues to concern itself with building out massive, do-it-all language models, niche and domain-specific models may be better equipped for specific jobs, said Bob Rogers, PhD, co-founder of BeeKeeperAI and CEO of Oii.ai.
Rather than focusing on a model that can act as a jack-of-all-trades, creating a small model that gets its information from a database of topic-specific information could lead to more accurate answers. “It’s an interesting effort to constrain a large language model to respect facts,” said Rogers. “This idea of making AI models that are more closely tuned to a knowledge base, that sticks to a certain set of facts, could be very appealing.”
These “pocket-sized models” suck up way less power than a large language model, said Rogers. For reference, OpenAI’s ChatGPT reportedly eats up 17,000 times more energy than the average US home consumes in a day.
Smaller, locally run models are also more secure, Rogers noted, as the entity that’s training the model knows exactly where the data is going. In the case of the Adobe patent, the person running the model may have control over the so-called knowledge base, rather than feeding data into the Big Tech AI machine. “If you can realistically run local models where you’re controlling where the data goes, that’s instantly a solution,” he said.
For Adobe’s purposes, this tech could add to its business of making AI easier to access for marketing and advertising, Rogers noted. The company’s patent activity is littered with AI innovations that fit this bill. The company also offers Firefly, its generative AI image model that it claims is commercially safe.
But this time, rather than tackling images, Adobe’s patent helps make AI-generated copy less bland and more specific, he noted. “I think that’s probably very valuable to Adobe in terms of selling tools that create useful advertising content,” Rogers said.