Microsoft Peels Back the Layers of its Deep Learning Models
Models that can explain how they draw their conclusions can help fight hallucination and build user trust.
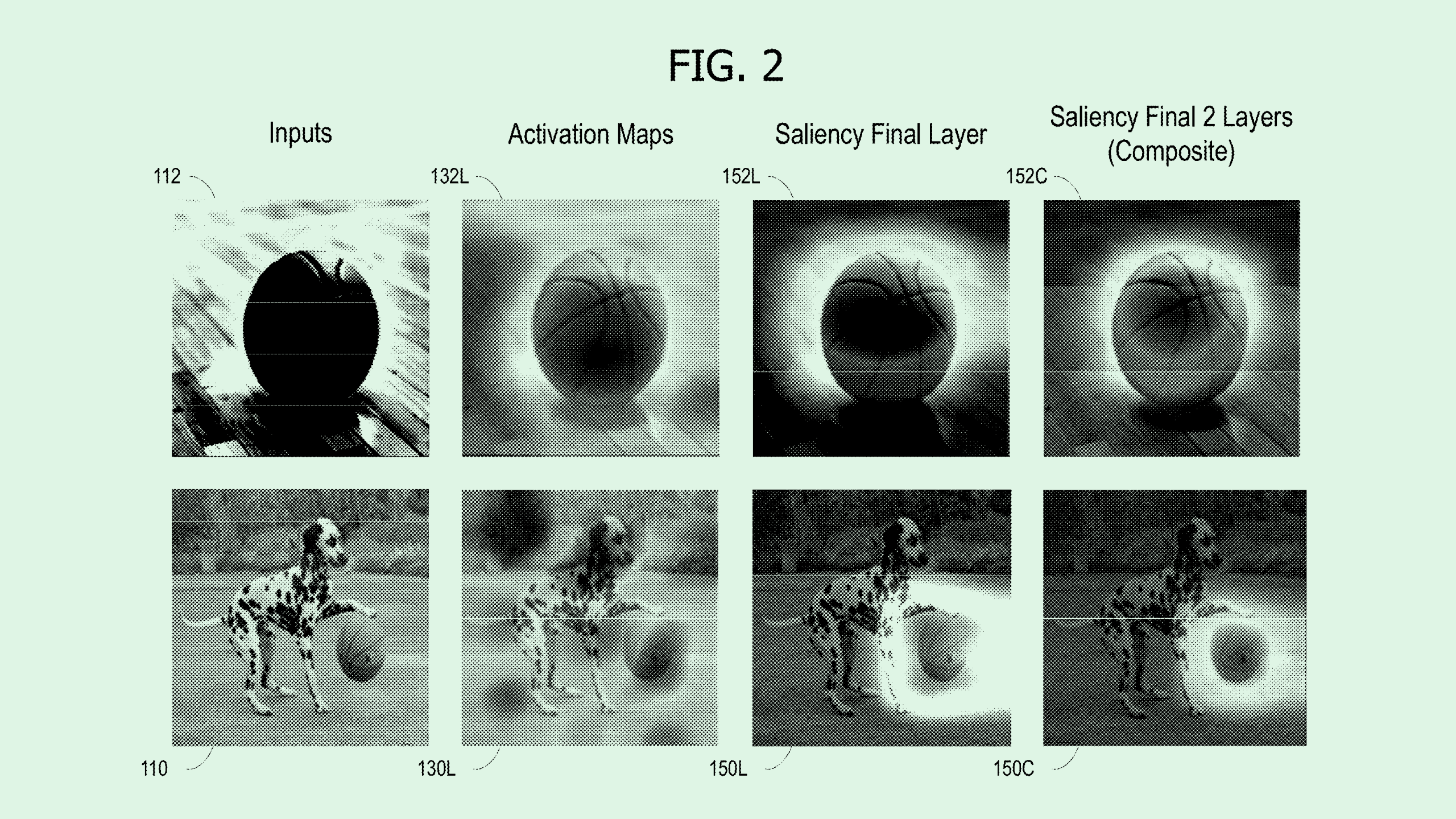
Sign up to uncover the latest in emerging technology.
Microsoft wants to dissect what goes on inside its AI models.
The company is seeking to patent a “diagnostic tool for deep learning similarity models.” Microsoft’s tech aims to provide insight into how neural networks, and more specifically image recognition and visual AI models, make their decisions.
“Because these are often deep learning models (e.g., 50 layers) that were trained using a series of labeled (tagged) images, ML-based decisions are largely viewed as “black box” processes,” Microsoft said in the filing. “As a result, decisions by ML models may be left unexplained.”
Microsoft’s system offers this insight by creating a “saliency map,” or a visual representation representing how a visual AI model is influenced by its input data.
First, an image-classifying neural network would do its job of recognizing and categorizing things within the photo. Once that’s complete, Microsoft’s tool kicks in, determining an “activation map” for a layer of the neural network. This activation map determines what regions of the image in particular triggered certain neurons in a neural network layer.
Then the tool creates a gradient map for that layer, which uses gradients to show how sensitive the model was to different parts of the image. By combining these two maps, the system creates a saliency map, which breaks down which parts of the image influence how a model decides to classify or recognize it.
For example, if the input image was a photo of a duck in a pond that was classified by the model as an image of a bird, a saliency map may look like a gradient circle surrounding the duck specifically, blurring out anything else in the image.
Finding ways for AI models to explain themselves could be crucial for figuring out where the error occurred when models spit out wrong answers or reproduce biases. By implementing mechanisms that let AI show its work, tech like this could allow developers to quickly diagnose issues and retrain the models to fix them. And since Microsoft’s patent is specifically aimed at visual models, this could help developers make facial recognition and computer vision more accurate.
Microsoft’s patent is just one of several from recent months that aims to demystify what goes on inside an AI model. Oracle has sought to patent “human understandable insights” for neural networks, Intel filed to patent a way to track and explain AI misuse, and even Boeing has filed an application for explainable AI in manufacturing. All of these filings convey a similar core issue across sectors: People generally don’t trust AI.
According to a global survey of 17,000 people on trust in AI from KPMG, more than 60% were wary about trusting AI models, and around 75% said they’d trust AI systems more if proper safeguards and oversights were in place. Creating and implementing more diagnostic tools for AI models could help build that trust.
Plus, while this patent is aimed at visual AI, explainability could also be pertinent for Microsoft, given that it and OpenAI are facing a legal battle with The New York Times due to copyright infringement, claiming the companies used its articles to train the large language models behind ChatGPT and Copilot, creating direct competition with the publication.
The Times’ case is one of many from writers, artists and creators that are going after AI firms over copyright claims. Creating AI models that can explain how they generate answers could allow AI companies to defend against allegations of copyright breaches – or reveal that they are, in fact, copycats.